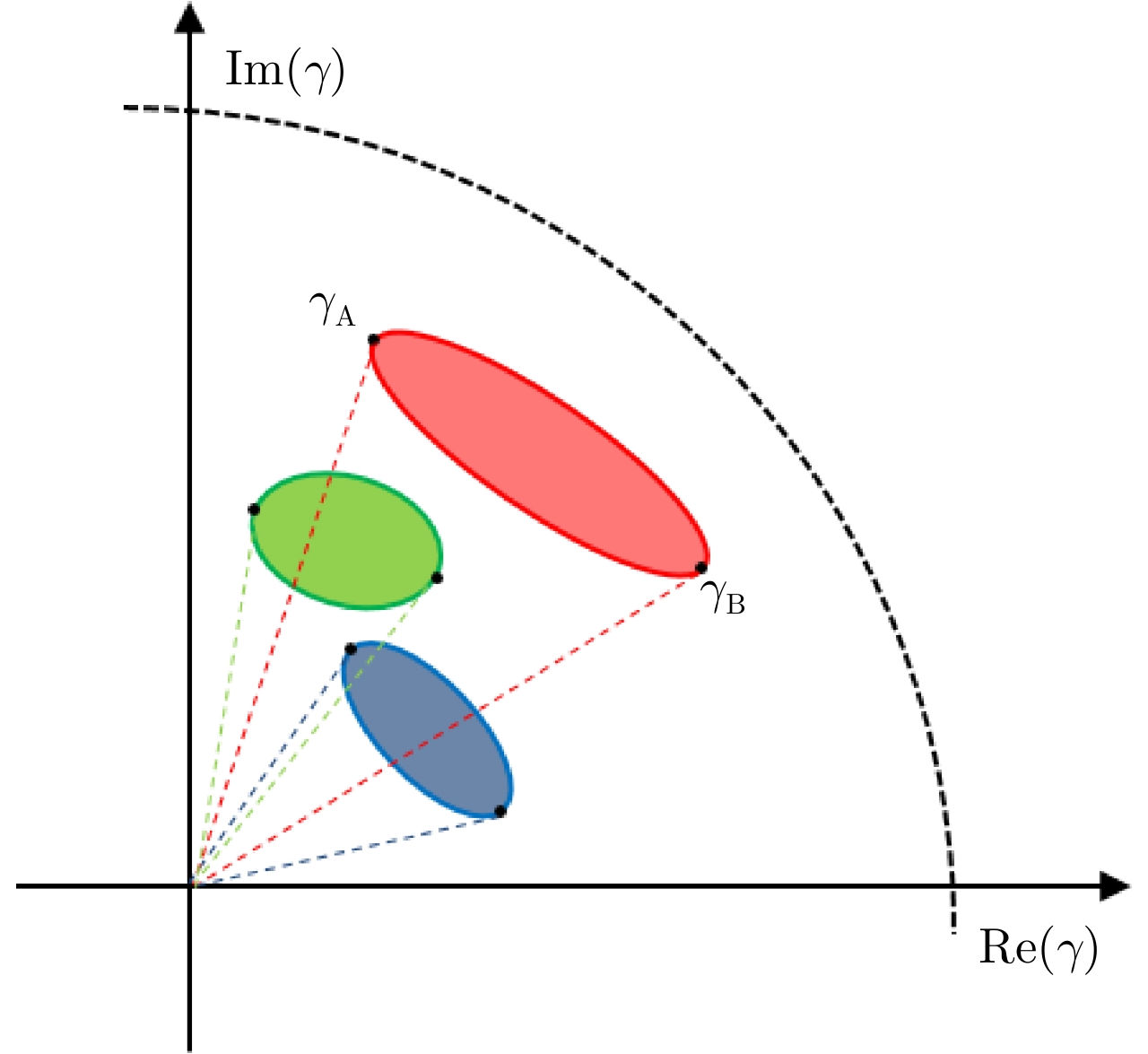
Citation: | CHEN Xiaolong, CHEN Weishi, RAO Yunhua, et al. Progress and prospects of radar target detection and recognition technology for flying birds and unmanned aerial vehicles[J]. Journal of Radars, 2020, 9(5): 803–827. doi: 10.12000/JR20068 |
森林高度是估算森林蓄积量及生物量的重要基础数据,对于研究森林资源状况以及分析全球生态环境、气候变化具有重要意义。极化合成孔径雷达干涉测量技术(Polarimetric SAR Interferometry, PolInSAR)采用微波监测模式,其回波信号不仅记录垂直结构及其属性信息,且可以区分同一分辨单元内不同散射体高度的能力,已被视为大范围、高分辨率、高精度反演森林高度的有效手段之一[1]。
为了实现利用PolInSAR观测量准确地提取森林高度,Thrauhft等人[2]建立了随机地体二层散射模型(Random Volume over Ground, RVoG),该模型将森林散射场景抽象为两层,即由随机均匀分布的散射体组成的植被层,以及微波信号不可穿透的地表层。随后,Papathanassiou等人[3,4]进一步分析PolInSAR复相干性与RVoG模型的关联,建立了利用PolInSAR反演森林高度的框架。实质上,该框架是基于体散射去相干的模型表达来反演森林高度等参数的,并且利用不同PolInSAR数据都获得了较高的反演精度[5-7]。
由于森林场景具有显著的时变性,具有长时间间隔的星载重轨干涉SAR(如ALOS-1至少为46天,ALOS-2为14天)散射场景内介电常数变化(如降雨)和风动都会产生严重的时间去相干。因此,除了体去相干的影响,时间去相干也是星载重轨极化干涉SAR数据中不可忽略的去相干因素,决定了森林参数反演的精度,甚至是反演成败的关键。为此,Yang等人[8]在随机移动散射模型(Random Motion over Ground, RMoG)[9]和体时去相干散射模型 (Volume Temporal Decorrelation, VTD)模型[10]基础上,提出一种时间去相干半经验森林高度反演方法。该方法结合少量机载LiDAR森林高度数据辅助时间去相干半经验模型解算,利用ALOS-1 PARSAR-1 HV极化相干幅度成功实现了大尺度森林高度反演。
然而,该方法需假设HV极化不包含地表散射回波能量贡献,事实上,L波段SAR信号具有较强的穿透性,尤其当森林高度较低或密度较小时,HV极化方式会记录显著地表回波信号。此外,该方法只适用于单基线干涉数据,尚未考虑多基线条件下,如何充分利用观测几何的多样性提升反演结果的可靠性。因此本文的目的是针对上述反演方法的限制,利用ALOS-2 PARSAR-2多基线PolInSAR数据更为准确地提取森林高度。主要思路如下:首先利用相干最大分离算法(Maximum Coherence Difference, MCD)在极化空间内寻求具有最少地面散射能量贡献的极化方式,以获得更为纯净的森林冠层散射贡献。然后利用该极化方式的相干幅度,在少量森林高度地面调查数据辅助下基于时间去相干半经验模型进行森林高度反演。在此基础之上,结合多基线数据根据PolInSAR相干集在复数平面内的几何表达,甄选最优观测干涉数据的反演结果作为森林高度反演最终结果。
综合顾及垂直方向上散射体分布产生的体去相干、散射场景内介电特性改变和植被风动引起的时间去相干同时占主导地位,星载重轨PolInSAR复相干系数一般形式表示为[8]
γ(ω)=eiφ0⋅γvd⋅γv/m+γgd⋅μ(ω)1+μ(ω) | (1) |
式中,
γv/m=∫h0exp[−12(4πλ)2σ2r(z)]⋅f(z)⋅exp(ikzz)dz∫h0f(z)dz | (2) |
其中,
σr(z)=σrhrz | (3) |
式中,
为了解决上述模型过参数化问题,Yang等人[8]对散射场景做如下假设:(1) 散射场景内时间去相干与消光系数在空间上具有一致性;(2) HV极化方式具有较小地面散射能量贡献,可假定其地体幅度比
γ=Sscene⋅exp[−12(4πσrαλhr)2h2]≈Sscene⋅sinc(hCscene) | (4) |
其中,
Sscene=|γvd|; Cscene=λhr2π2σrα | (5) |
已有方法主要选用对森林冠层较为敏感的HV极化方式进行模型求解,但是ALOS-2 PALSAR-2发射具有较强穿透能力的L波段电磁波,HV极化方式回波信号中同样会记录显著地表回波信号。鉴于此,本文利用ALOS-2 PALSAR-2全极化数据结合极化相干最优理论,尽可能抑制地表回波信号的干扰。具体方法如下:
在主辅极化SAR影像散射机制相同的情况下,极化干涉SAR的复相干系数表示为[12]
γ=⟨ωHΩ12ω⟩√⟨ωHT11ω⟩⟨ωHT22ω⟩ | (6) |
式中,自相关矩阵
Re(γeiϕ)=ωHAωωHTωA=eiϕΩ12+e−iϕΩH122,T=T11+T222} | (7) |
式中,
γ1=ωH1Ω12ω1ωH1Tω1, γ2=ωH2Ω12ω2ωH2Tω2 | (8) |
相比传统InSAR技术只能获取HH, HV或VV极化方式对应的复相干系数,相干集中包含了特定极化散射机理对应的复相干系数,为寻求极化空间内具有更为纯净森林冠层散射贡献的极化方式提供了可能。相干区域范围示意如图1所示,其中在相干区域成对边界点中距离最远的一对相干系数点
kz>0:ifarg(γAγ∗B)>0thenγ(μmin)=γA,γ(μmax)=γBifarg(γAγ∗B)<0thenγ(μmin)=γB,γ(μmax)=γAkz<0:ifarg(γAγ∗B)<0thenγ(μmin)=γA,γ(μmax)=γBifarg(γAγ∗B)>0thenγ(μmin)=γB,γ(μmax)=γA} | (9) |
其中,
kz=4πB⊥λRsinθ | (10) |
即便简化了模型参数和采用多基线PolInSAR数据增加了观测量,利用传统多维非线性迭代求解时间去相干半经验模型仍存在秩亏问题。因此本文采用一种外部数据辅助反演法[8],即先利用小范围真实森林高度数据辅助解算出模型参数
主成分分析思想[17]为实现上述思路提供了契机,即通过对训练数据中
X=[Var(hreal)Cov(hreal,hinvert)Cov(hinvert,hreal)Var(hinvert)]=[P11P12P21P22][λ100λ2][P11P12P21P22]−1k=P21P11} | (11) |
其中
b=M(hreal)−M(hinvert)[M(hreal)+M(hinvert)]/2 | (12) |
式中,M 表示取平均运算。
散点点阵椭圆主轴确定后,显然可以通过建立使逼近参数
(k−1)2+(b−0)2=min | (13) |
该目标函数可以利用高斯-牛顿迭代算法进行非线性最小二乘求解,如式(14)所示
[S∗sceneC∗scene]=(JT0J0)−1JT0[1−k00−b0]+[Sscene0Cscene0] | (14) |
式中,
J0=[∂k∂Sscene∂k∂Cscene∂b∂Sscene∂b∂Cscene]|Sscene0Cscene0 | (15) |
然后将得到修正后的模型参数作为新的初始点进行下一次迭代,经过多次迭代后即可获得最佳模型参数
|[S∗sceneC∗scene]−[S(∗−1)sceneC(∗−1)scene]|<ε | (16) |
在利用训练数据求得时间去相干半经验模型参数后,对每个像元求解一元非线性方程得到整个散射场景内的森林高度结果。
时间去相干、体去相干以及其他噪声等因素会共同影响PolInSAR复相干性在复平面单位圆上的几何表达[18]。在多基线配置下,不同干涉对在同一分辨单元内往往呈现出不同的相干区域结构(如图1所示)。而相干特性
P=|γ(μmin)−γ(μmax)||γ(μmin)+γ(μmax)| | (17) |
式中,
通过相干特性指标P 甄选出不同干涉对在同一分辨单元内反演出的最优森林高度值作为多基线PolInSAR森林高度融合结果,多基线PolInSAR融合反演框架可表示为
max‖P1(γ1(μmin),γ1(μmax))P2(γ2(μmin),γ2(μmax))⋮PN(γN(μmin),γN(μmax))‖ | (18) |
式中,N 为极化干涉SAR观测基线数。
研究区域黄丰桥国有林场(27°
地面实测数据由中南林业科技大学于2016年6~7月采集得到,通过在林区范围内选取60个相互独立的林分样地以确保避免空间自相关,每个林分样地规格为30×30 m。树高则基于单木测高原理利用激光测高仪测得,林分高度范围为4.60~20.20 m,平均高度为13.24 m。本文通过随机采样,将60个林分样地数据随机分为45个训练数据(图3黄点所示)和15个验证数据(图3红点所示)两组。
多基线星载重轨PolInSAR数据是利用日本宇航局(JAXA)提供的5景覆盖研究区域的ALOS-2 PALSAR-2 L波段全极化数据。该SAR影像范围如图3蓝色虚线所示,获取时间为2016年6月至8月,获取模式为StripMap2(SM2),影像主要参数信息如表1所示。将5景SAR影像组成3个时间基线为14天的干涉影像对(BL1, BL2, BL3),然后各自进行配准处理,并进行公共带通滤波以确保去除几何去相干。相干性以11×11窗口进行估计,并应用Boxcar滤波进行平滑处理以消除斑点效应。最后利用SRTM DEM对SAR影像进行地理编码,并将其重采样至与DEM空间分辨率一致(30×30 m)。图4为3个干涉对的HV极化和
日期(2016年) | 垂直有效波数(rad/m) | 时间基线(天) | 距离向/方位向分辨率(m) | 中心入射角 (°) | 极化方式 |
0616—0630 (BL1) | 0.013~0.015 | ||||
0630—0714 (BL2) | 0.010~0.011 | 14 | 2.86/2.97 | 38.99 | Full |
0811—0825 (BL3) | 0.009~0.010 |
以选取的15个验证林分的实测森林高度(H-field)对反演结果(H-invert)进行分析评价,图5为3个干涉对利用HV极化反演得到的散点图结果,均方根误差RMSE分别为:4.20 m, 4.03 m和3.42 m。利用
从上述单基线森林高度反演结果看,不同干涉对反演整体精度较为接近,但是对于同一林分在利用不同干涉对反演的结果却存在明显差异。因此,当多基线数据可用时,我们进一步在单基线PolInSAR森林高度反演结果的基础上挖掘PolInSAR数据本身特性并对其森林高度反演能力进行评判。与时间去相干相关的参数
模型参数 | BL1 | BL2 | BL3 |
Sscene | 0.69 | 0.78 | 0.78 |
Cscene | 9.88 | 10.08 | 11.14 |
3个干涉对在验证林分的相干特性P值、反演森林高度值以及多基线融合森林高度值如表3所示,从整体看,根据相干特性P值大小从3个单基线PolInSAR反演结果中甄选出的森林高度结果更接近于实测真实森林高度。整个实验区的多基线PolInSAR融合反演结果以及精度评定如图7所示,均方根误差RMSE为2.05 m,相比于已有的方法,本文提出的多基线PolInSAR融合反演策略精度至少提高了40%(与图5中BL3基线结果对比),同时,相关系数也提升至0.81。
林分样地编号 | BL1 P值 / 森林高度(m) | BL2 P值 / 森林高度(m) | BL3 P值 / 森林高度(m) | 多基线融合结果(m) | 实测森林高度(m) |
1 | 0.130 / 17.82 | 0.113 / 17.02 | 0.081 / 16.89 | 17.82 | 14.43 |
2 | 0.116 / 14.38 | 0.104 / 15.30 | 0.091 / 16.52 | 14.38 | 14.20 |
3 | 0.092 / 12.46 | 0.075 / 15.83 | 0.135 / 11.34 | 11.34 | 9.80 |
4 | 0.103 / 15.21 | 0.111 / 15.34 | 0.119 / 14.19 | 14.19 | 16.00 |
5 | 0.106 / 6.86 | 0.106 / 7.24 | 0.131 / 8.31 | 8.31 | 10.70 |
6 | 0.110 / 12.98 | 0.083 / 14.67 | 0.118 / 11.89 | 11.89 | 13.50 |
7 | 0.114 / 13.35 | 0.096 / 15.30 | 0.101 / 16.10 | 13.35 | 13.43 |
8 | 0.079 / 14.29 | 0.106 / 16.15 | 0.117 / 16.22 | 16.22 | 16.95 |
9 | 0.069 / 12.12 | 0.090 / 17.63 | 0.060 / 12.30 | 17.63 | 20.10 |
10 | 0.104 / 12.33 | 0.089 / 13.67 | 0.102 / 11.72 | 12.33 | 15.60 |
11 | 0.075 / 18.34 | 0.103 / 16.75 | 0.154 / 10.16 | 10.16 | 13.30 |
12 | 0.113 / 9.08 | 0.134 / 9.46 | 0.106 / 12.69 | 9.46 | 11.00 |
13 | 0.086 / 13.76 | 0.096 / 9.07 | 0.109 / 16.00 | 16.00 | 16.40 |
14 | 0.197 / 10.17 | 0.230 / 8.71 | 0.186 / 9.51 | 8.71 | 6.00 |
15 | 0.103 / 14.59 | 0.064 / 19.17 | 0.128 / 15.40 | 15.40 | 14.70 |
在多基线全极化数据可用条件下,弥补单基线InSAR观测信息不足以及几何结构单一的问题,对于反演结果整体精度提升具有重要作用。本文提出了一种星载重轨多基线PolInSAR反演森林高度的策略,对InSAR极化空间和观测几何空间进行扩展,主要结论如下:
(1) 该方法利用MCD相干优化算法获得对体散射最为敏感的极化方式,并基于时间去相干半经验模型进行森林高度反演,使每条单基线反演精度在一定程度上都有所提高。
(2) 利用由相干特性指标P确定的相干区域最优准则可以优选出同一分辨单元内最优的单基线森林高度反演结果。因此,相比仅利用单基线单一极化反演方法,多基线PolInSAR融合策略具有更好的稳定性,精度也更高。
[1] |
王维, 项洪达. 鸟击风险计算方法研究[J]. 中国民航大学学报, 2019, 37(5): 21–24.
WANG Wei and XIANG Hongda. Calculation method of aircraft bird strike risk[[J]. Journal of Civil Aviation University of China, 2019, 37(5): 21–24.
|
[2] |
于飞, 刘东华, 贺飞扬. 无人机“黑飞”对电磁空间安全的挑战[J]. 中国无线电, 2018, (8): 43–44. doi: 10.3969/j.issn.1672-7797.2018.08.026
YU Fei, LIU Donghua, and HE Feiyang. UAV “black flying” challenges the safety of electromagnetic space[J]. China Radio, 2018, (8): 43–44. doi: 10.3969/j.issn.1672-7797.2018.08.026
|
[3] |
陈唯实. 轻小型无人机监管、探测与干扰技术[J]. 中国民用航空, 2017, (7): 33–34.
CHEN Weishi. The supervision, detection and jamming technologies for light and small UAS[J]. China Civil Aviation, 2017, (7): 33–34.
|
[4] |
吴小松, 房之军, 陈通海. 民用无人机反制技术研究[J]. 中国无线电, 2018, (3): 55–58. doi: 10.3969/j.issn.1672-7797.2018.03.038
WU Xiaosong, FANG Zhijun, and CHEN Tonghai. Research on civil UAV countermeasure technology[J]. China Radio, 2018, (3): 55–58. doi: 10.3969/j.issn.1672-7797.2018.03.038
|
[5] |
罗淮鸿, 卢盈齐. 国外反“低慢小”无人机能力现状与发展趋势[J]. 飞航导弹, 2019, (6): 32–36. doi: 10.16338/j.issn.1009-1319.20180472
LUO Huaihong and LU Yingqi. Ability status and development trend of anti-“low, slow and small” UAVs[J]. Aerodynamic Missile Journal, 2019, (6): 32–36. doi: 10.16338/j.issn.1009-1319.20180472
|
[6] |
陈小龙, 关键, 黄勇, 等. 雷达低可观测目标探测技术[J]. 科技导报, 2017, 35(11): 30–38. doi: 10.3981/j.issn.1000-7857.2017.11.004
CHEN Xiaolong, GUAN Jian, HUANG Yong, et al. Radar low-observable target detection[J]. Science&Technology Review, 2017, 35(11): 30–38. doi: 10.3981/j.issn.1000-7857.2017.11.004
|
[7] |
陈小龙, 关键, 黄勇, 等. 雷达低可观测动目标精细化处理及应用[J]. 科技导报, 2017, 35(20): 19–27. doi: 10.3981/j.issn.1000-7857.2017.20.002
CHEN Xiaolong, GUAN Jian, HUANG Yong, et al. Radar refined processing and its applications for low-observable moving target[J]. Science&Technology Review, 2017, 35(20): 19–27. doi: 10.3981/j.issn.1000-7857.2017.20.002
|
[8] |
NOHARA T J, ENG B, ENG M, et al. An overview of avian radar developments - past, present and future[C]. The 2007 Bird Strike Committee USA/Canada, 9th Annual Meeting, Kingston, Ontario, 2007.
|
[9] |
BEASON R C, NOHARA T J, and WEBER P. Beware the Boojum: Caveats and strengths of avian radar[J]. Human-Wildlife Interactions, 2013, 7(1): 16–46.
|
[10] |
BRAUN C E. Techniques for wildlife investigations and management[J]. The Condor, 2007, 109(4): 981–983. doi: 10.1093/condor/109.4.981
|
[11] |
宁焕生, 刘文明, 李敬, 等. 航空鸟击雷达鸟情探测研究[J]. 电子学报, 2006, 34(12): 2232–2237. doi: 10.3321/j.issn:0372-2112.2006.12.023
NING Huansheng, LIU Wenming, LI Jing, et al. Research on radar avian detection for aviation[J]. Acta Electronica Sinica, 2006, 34(12): 2232–2237. doi: 10.3321/j.issn:0372-2112.2006.12.023
|
[12] |
陈唯实, 宁焕生, 刘文明, 等. 基于雷达图像的飞鸟目标检测与信息提取[J]. 系统工程与电子技术, 2008, 30(9): 1624–1627. doi: 10.3321/j.issn:1001-506X.2008.09.006
CHEN Weishi, NING Huansheng, LIU Wenming, et al. Flying bird targets detection and information extraction based on radar images[J]. Systems Engineering and Electronics, 2008, 30(9): 1624–1627. doi: 10.3321/j.issn:1001-506X.2008.09.006
|
[13] |
陈唯实, 李敬. 雷达探鸟技术发展与应用综述[J]. 现代雷达, 2017, 39(2): 7–17. doi: 10.16592/j.cnki.1004-7859.2017.02.002
CHEN Weishi and LI Jing. Review on development and applications of avian radar technology[J]. Modern Radar, 2017, 39(2): 7–17. doi: 10.16592/j.cnki.1004-7859.2017.02.002
|
[14] |
陈唯实, 万显荣, 李敬. 机场净空区非合作无人机目标探测技术[J]. 民航学报, 2018, 2(5): 54–57, 45. doi: 10.3969/j.issn.2096-4994.2018.05.014
CHEN Weishi, WAN Xianrong, and LI Jing. Detection technology of non-cooperative UAV targets in airport clearance area[J]. Journal of Civil Aviation, 2018, 2(5): 54–57, 45. doi: 10.3969/j.issn.2096-4994.2018.05.014
|
[15] |
蔡亚梅, 姜宇航, 赵霜. 国外反无人机系统发展动态与趋势分析[J]. 航天电子对抗, 2017, 33(2): 59–64. doi: 10.16328/j.htdz8511.2017.02.016
CAI Yamei, JIANG Yuhang, and ZHAO Shuang. Development status and trend analysis of counter UAV systems[J]. Aerospace Electronic Warfare, 2017, 33(2): 59–64. doi: 10.16328/j.htdz8511.2017.02.016
|
[16] |
罗德与施瓦茨(中国)科技有限公司. 无人机自动识别、定位和压制系统(一)[J]. 中国无线电, 2016, (8): 72–73. doi: 10.3969/j.issn.1672-7797.2016.08.047
Rohde & Schwarz (China) Technology Co., L td. UAV automatic identification, positioning and suppression system (One)[J]. China Radio, 2016, (8): 72–73. doi: 10.3969/j.issn.1672-7797.2016.08.047
|
[17] |
罗德与施瓦茨(中国)科技有限公司. 无人机自动识别、定位和压制系统(二)[J]. 中国无线电, 2016, (9): 71–72. doi: 10.3969/j.issn.1672-7797.2016.09.045
Rohde & Schwarz (China) Technology Co., L td. UAV automatic identification, positioning and suppression system (Two)[J]. China Radio, 2016, (9): 71–72. doi: 10.3969/j.issn.1672-7797.2016.09.045
|
[18] |
罗德与施瓦茨(中国)科技有限公司. 无人机自动识别、定位和压制系统(三)[J]. 中国无线电, 2016, (10): 72–73. doi: 10.3969/j.issn.1672-7797.2016.10.044
Rohde & Schwarz (China) Technology Co., L td. UAV automatic identification, positioning and suppression system (Three)[J]. China Radio, 2016, (10): 72–73. doi: 10.3969/j.issn.1672-7797.2016.10.044
|
[19] |
JEON S, SHIN J W, LEE Y J, et al. Empirical study of drone sound detection in real-life environment with deep neural networks[C]. The 2017 25th European Signal Processing Conference, Kos, Greece, 2017. doi: 10.23919/EUSIPCO.2017.8081531.
|
[20] |
HOMMES A, SHOYKHETBROD A, NOETEL D, et al. Detection of acoustic, electro-optical and RADAR signatures of small unmanned aerial vehicles[C]. SPIE 9997, Target and Background Signatures Ⅱ, Edinburgh, USA, 2016. doi: 10.1117/12.2242180.
|
[21] |
MEZEI J and MOLNÁR A. Drone sound detection by correlation[C]. The 2016 IEEE 11th International Symposium on Applied Computational Intelligence and Informatics, Timisoara, Romania, 2016. doi: 10.1109/SACI.2016.7507430.
|
[22] |
陈超帅, 王世勇. 大疆无人机目标红外辐射特性测量及温度反演[J]. 光电工程, 2017, 44(4): 427–434. doi: 10.3969/j.issn.1003-501X.2017.04.007
CHEN Chaoshuai and WANG Shiyong. Infrared radiation characteristics measurement and temperature retrieval based on DJI unmanned aerial vehicle[J]. Opto-Electronic Engineering, 2017, 44(4): 427–434. doi: 10.3969/j.issn.1003-501X.2017.04.007
|
[23] |
郭溪溪. 低空慢速小目标检测识别与威胁度评估[D]. [硕士论文], 中国科学院长春光学精密机械与物理研究所, 2017.
GUO Xixi. Detection and recognition and thread assessment for small target at low altitude and slow speed[D]. [Master dissertation], Changchun Institute of Optics, Fine Mehcanics and Physics, Chinese Academy of Sciences, 2017.
|
[24] |
MÜLLER T. Robust drone detection for day/night counter-UAV with static VIS and SWIR cameras[C]. SPIE 10190, Ground/Air Multisensor Interoperability, Integration, and Networking for Persistent ISR VⅢ, Anaheim, USA, 2017. doi: 10.1117/12.2262575.
|
[25] |
KLARE J, BIALLAWONS O, and CERUTTI-MAORI D. Detection of UAVs using the MIMO radar MIRA-CLE Ka[C]. The EUSAR 2016: 11th European Conference on Synthetic Aperture Radar, Hamburg, Germany, 2016.
|
[26] |
WILLIAMS H. AUDS to target small unmanned intruders[J]. Jane’s International Defense Review, 2015, 48: 22.
|
[27] |
郭珊珊. 反无人机技术与产品发展现状[J]. 军事文摘, 2016, (19): 36–39.
GUO Shanshan. Anti-UAV technology and product development status[J]. Military Digest, 2016, (19): 36–39.
|
[28] |
CLEMENTE C and SORAGHAN J J. Passive bistatic radar for helicopters classification: A feasibility study[C]. 2012 IEEE Radar Conference, Atlanta, USA, 2012. doi: 10.1109/RADAR.2012.6212273.
|
[29] |
LIU Yuqi, WAN Xianrong, TANG Hui, et al. Digital television based passive bistatic radar system for drone detection[C]. 2017 IEEE Radar Conference, Seattle, USA, 2017. doi: 10.1109/RADAR.2017.7944443.
|
[30] |
SCHÜPbach C, PATRY C, MAASDORP F, et al. Micro-UAV detection using DAB-based passive radar[C]. 2017 IEEE Radar Conference, Seattle, USA, 2017. doi: 10.1109/RADAR.2017.7944357.
|
[31] |
TIKKINEN J, HILTUNEN K, MARTIKAINEN K, et al. Helicopter detection capability of passive coherent location (PCL) radar[C]. The 2012 9th European Radar Conference, Amsterdam, Netherlands, 2012.
|
[32] |
TIKKINEN J, HILTUNEN K, and MARTIKAINEN K. Utilization of long coherent integration time in helicopter recognition by passive coherent location (PCL) radar[C]. 2013 European Radar Conference, Nuremberg, Germany, 2013.
|
[33] |
BĄCZYK M K, MISIUREWICZ J, GROMEK D, et al. Analysis of recorded helicopter echo in a passive bistatic radar[C]. 2013 European Radar Conference, Nuremberg, Germany, 2013.
|
[34] |
MAASDORP F D V, CILLIERS J E, INGGS M R, et al. Simulation and measurement of propeller modulation using FM broadcast band commensal radar[J]. Electronics Letters, 2013, 49(23): 1481–1482. doi: 10.1049/el.2013.2875
|
[35] |
MARTELLI T, COLONE F, and CARDINALI R. DVB-T based passive radar for simultaneous counter-drone operations and civil air traffic surveillance[J]. IET Radar,Sonar&Navigation, 2020, 14(4): 505–515. doi: 10.1049/iet-rsn.2019.0309
|
[36] |
MARTELLI T, MURGIA F, COLONE F, et al. Detection and 3D localization of ultralight aircrafts and drones with a WiFi-based passive radar[C]. International Conference on Radar Systems, Belfast, UK, 2017. doi: 10.1049/cp.2017.0423
|
[37] |
MUSA S A, RSA R A, SALI A, et al. DVBS based forward scattering radar for drone detection[C]. The 2019 20th International Radar Symposium, Ulm, Germany, 2019. doi: 10.23919/IRS.2019.8767456.
|
[38] |
LIU Yuqi, YI Jianxin, WAN Xianrong, et al. Time varying clutter suppression in CP OFDM based passive radar for slowly moving targets detection[J]. IEEE Sensors Journal, 2020, in press. doi: 10.1109/JSEN.2020.2986717
|
[39] |
刘玉琪, 易建新, 万显荣, 等. 数字电视外辐射源雷达多旋翼无人机微多普勒效应实验研究[J]. 雷达学报, 2018, 7(5): 585–592. doi: 10.12000/JR18062
LIU Yuqi, YI Jianxin, WAN Xianrong, et al. Experimental research on micro-Doppler effect of multi-rotor drone with digital television based passive radar[J]. Journal of Radars, 2018, 7(5): 585–592. doi: 10.12000/JR18062
|
[40] |
YI Yucheng, WAN Xianrong, YI Jianxin, et al. polarization diversity technology research in passive radar based on subcarrier processing[J]. IEEE Sensors Journal, 2019, 19(5): 1710–1719. doi: 10.1109/JSEN.2018.2881226
|
[41] |
DAN Yangpeng, YI Jianxin, WAN Xianrong, et al. LTE-based passive radar for drone detection and its experimental results[J]. The Journal of Engineering, 2019, 2019(20): 6910–6913. doi: 10.1049/joe.2019.0583
|
[42] |
PATEL J S, FIORANELLI F, and ANDERSON D. Review of radar classification and RCS characterisation techniques for small UAVs or drones[J]. IET Radar,Sonar&Navigation, 2018, 12(9): 911–919. doi: 10.1049/iet-rsn.2018.0020
|
[43] |
KNOEDLER B, ZEMMARI R, and KOCH W. On the detection of small UAV using a GSM passive coherent location system[C]. The 2016 17th International Radar Symposium, Krakow, Poland, 2016. doi: 10.1109/IRS.2016.7497375.
|
[44] |
POULLIN D. UAV detection and localization using passive DVB-T radar MFN and SFN[R]. 2016.
|
[45] |
HOFFMANN F, RITCHIE M, FIORANELLI F, et al. Micro-Doppler based detection and tracking of UAVs with multistatic radar[C]. 2016 IEEE Radar Conference, Philadelphia, USA, 2016. doi: 10.1109/RADAR.2016.7485236.
|
[46] |
JAHANGIR M, BAKER C J, and OSWALD G A. Doppler characteristics of micro-drones with L-Band multibeam staring radar[C]. 2017 IEEE Radar Conference, Seattle, USA, 2017. doi: 10.1109/RADAR.2017.7944360.
|
[47] |
张群, 胡健, 罗迎, 等. 微动目标雷达特征提取、成像与识别研究进展[J]. 雷达学报, 2018, 7(5): 531–547. doi: 10.12000/JR18049
ZHANG Qun, HU Jian, LUO Ying, et al. Research progresses in radar feature extraction, imaging, and recognition of target with micro-motions[J]. Journal of Radars, 2018, 7(5): 531–547. doi: 10.12000/JR18049
|
[48] |
陈小龙, 关键, 何友. 微多普勒理论在海面目标检测中的应用及展望[J]. 雷达学报, 2013, 2(1): 123–134. doi: 10.3724/SP.J.1300.2013.20102
CHEN Xiaolong, GUAN Jian, and HE You. Applications and prospect of micro-motion theory in the detection of sea surface target[J]. Journal of Radars, 2013, 2(1): 123–134. doi: 10.3724/SP.J.1300.2013.20102
|
[49] |
刘凯越, 张晨新, 刘刚, 等. 关于飞机雷达探测飞鸟的RCS建模仿真[J]. 计算机仿真, 2016, 33(5): 120–124, 228. doi: 10.3969/j.issn.1006-9348.2016.05.025
LIU Kaiyue, ZHANG Chenxin, LIU Gang, et al. Modeling and simulation about RCS of aircraft radar detecting bird[J]. Computer Simulation, 2016, 33(5): 120–124, 228. doi: 10.3969/j.issn.1006-9348.2016.05.025
|
[50] |
SINGH A K and KIM Y H. Automatic measurement of blade length and rotation rate of drone using w-band micro-Doppler radar[J]. IEEE Sensors Journal, 2018, 18(5): 1895–1902. doi: 10.1109/JSEN.2017.2785335
|
[51] |
宋晨, 周良将, 吴一戎, 等. 基于自相关-倒谱联合分析的无人机旋翼转动频率估计方法[J]. 电子与信息学报, 2019, 41(2): 255–261. doi: 10.11999/JEIT180399
SONG Chen, ZHOU Liangjiang, WU Yirong, et al. An estimation method of rotation frequency of unmanned aerial vehicle based on auto-correlation and cepstrum[J]. Journal of Electronics&Information Technology, 2019, 41(2): 255–261. doi: 10.11999/JEIT180399
|
[52] |
陈永彬, 李少东, 杨军, 等. 一种旋翼叶片微动特征提取新方法[J]. 雷达科学与技术, 2017, 15(1): 13–18, 28. doi: 10.3969/j.issn.1672-2337.2017.01.003
CHEN Yongbin, LI Shaodong, YANG Jun, et al. A new method for micro-motion signature extraction of rotor blades[J]. Radar Science and Technology, 2017, 15(1): 13–18, 28. doi: 10.3969/j.issn.1672-2337.2017.01.003
|
[53] |
JAHANGIR M and BAKER C J. Extended dwell Doppler characteristics of birds and micro-UAS at l-band[C]. The 2017 18th International Radar Symposium, Prague, Czech Republic, 2017: 1–10. doi: 10.23919/IRS.2017.8008144.
|
[54] |
RAHMAN S and ROBERTSON D A. Radar micro-Doppler signatures of drones and birds at K-band and W-band[J]. Scientific Reports, 2018, 8(1): 17396. doi: 10.1038/s41598-018-35880-9
|
[55] |
CHEN Xiaolong, GUAN Jian, LIU Ningbo, et al. Maneuvering target detection via radon-fractional Fourier transform-based long-time coherent integration[J]. IEEE Transactions on Signal Processing, 2014, 62(4): 939–953. doi: 10.1109/TSP.2013.2297682
|
[56] |
关键, 陈小龙, 于晓涵. 雷达高速高机动目标长时间相参积累检测方法[J]. 信号处理, 2017, 33(3A): 1–8. doi: 10.16798/j.issn.1003-0530.2017.3A.001
GUAN Jian, CHEN Xiaolong, and YU Xiaohan. Long-time coherent integration-based detection method for high-speed and highly maneuvering radar target[J]. Journal of Signal Processing, 2017, 33(3A): 1–8. doi: 10.16798/j.issn.1003-0530.2017.3A.001
|
[57] |
LI Xiaolong, CUI Guolong, YI Wei, et al. Radar maneuvering target detection and motion parameter estimation based on TRT-SGRFT[J]. Signal Processing, 2017, 133: 107–116. doi: 10.1016/j.sigpro.2016.10.014
|
[58] |
CHEN Xiaolong, GUAN Jian, CHEN Weishi, et al. Sparse long-time coherent integration-based detection method for radar low-observable manoeuvring target[J]. IET Radar,Sonar&Navigation, 2020, 14(4): 538–546. doi: 10.1049/iet-rsn.2019.0313
|
[59] |
DJUROVIĆ I and SIMEUNOVIĆ M. Parameter estimation of 2D polynomial phase signals using NU sampling and 2D CPF[J]. IET Signal Processing, 2018, 12(9): 1140–1145. doi: 10.1049/iet-spr.2018.5083
|
[60] |
CHEN Xiaolong, GUAN Jian, WANG Guoqing, et al. Fast and refined processing of radar maneuvering target based on hierarchical detection via sparse fractional representation[J]. IEEE Access, 2019, 7: 149878–149889. doi: 10.1109/ACCESS.2019.2947169
|
[61] |
罗迎, 张群, 封同安, 等. OFD-LFM MIMO雷达中旋转目标微多普勒效应分析及三维微动特征提取[J]. 电子与信息学报, 2011, 33(1): 8–13. doi: 10.3724/SP.J.1146.2010.00234
LUO Ying, ZHANG Qun, FENG Tongan, et al. Micro-Doppler effect analysis of rotating target and three-dimensional micro-motion feature extraction in OFD-LFM MIMO radar[J]. Journal of Electronics&Information Technology, 2011, 33(1): 8–13. doi: 10.3724/SP.J.1146.2010.00234
|
[62] |
赵双, 鲁卫红, 冯存前, 等. 多视角微多普勒融合的进动目标特征提取[J]. 信号处理, 2016, 32(3): 296–303. doi: 10.16798/j.issn.1003-0530.2016.03.006
ZHAO Shuang, LU Weihong, FENG Cunqian, et al. Feature extraction of precession targets based on multi-aspect micro-Doppler fusion[J]. Journal of Signal Processing, 2016, 32(3): 296–303. doi: 10.16798/j.issn.1003-0530.2016.03.006
|
[63] |
RITCHIE M, FIORANELLI F, BORRION H, et al. Multistatic micro-Doppler radar feature extraction for classification of unloaded/loaded micro-drones[J]. IET Radar,Sonar&Navigation, 2017, 11(1): 116–124. doi: 10.1049/iet-rsn.2016.0063
|
[64] |
万显荣, 易建新, 程丰, 等. 单频网分布式外辐射源雷达技术[J]. 雷达学报, 2014, 3(6): 623–631. doi: 10.12000/JR14156
WAN Xianrong, YI Jianxin, CHENG Feng, et al. Single frequency network based distributed passive radar technology[J]. Journal of Radars, 2014, 3(6): 623–631. doi: 10.12000/JR14156
|
[65] |
万显荣, 孙绪望, 易建新, 等. 分布式数字广播电视外辐射源雷达系统同步设计与测试[J]. 雷达学报, 2017, 6(1): 65–72. doi: 10.12000/JR16134
WAN Xianrong, SUN Xuwang, YI Jianxin, et al. Synchronous design and test of distributed passive radar systems based on digital broadcasting and television[J]. Journal of Radars, 2017, 6(1): 65–72. doi: 10.12000/JR16134
|
[66] |
CHENG Gong, HAN Junwei, and LU Xiaoqiang. Remote sensing image scene classification: Benchmark and state of the art[J]. Proceedings of the IEEE, 2017, 105(10): 1865–1883. doi: 10.1109/JPROC.2017.2675998
|
[67] |
文贡坚, 朱国强, 殷红成, 等. 基于三维电磁散射参数化模型的SAR目标识别方法[J]. 雷达学报, 2017, 6(2): 115–135. doi: 10.12000/JR17034
WEN Gongjian, ZHU Guoqiang, YIN Hongcheng, et al. SAR ATR Based on 3D parametric electromagnetic scattering model[J]. Journal of Radars, 2017, 6(2): 115–135. doi: 10.12000/JR17034
|
[68] |
OH B S, GUO Xin, WAN Fangyuan, et al. Micro-Doppler mini-UAV classification using empirical-mode decomposition features[J]. IEEE Geoscience and Remote Sensing Letters, 2018, 15(2): 227–231. doi: 10.1109/LGRS.2017.2781711
|
[69] |
BIRTCHER C R, BALANIS C A, and DECARLO D. Rotor-blade modulation on antenna amplitude pattern and polarization: Predictions and measurements[J]. IEEE Transactions on Electromagnetic Compatibility, 1999, 41(4): 384–393. doi: 10.1109/15.809828
|
[70] |
ZHAO Yichao and SU Yi. Synchrosqueezing phase analysis on micro-Doppler parameters for small UAVs identification with multichannel radar[J]. IEEE Geoscience and Remote Sensing Letters, 2020, 17(3): 411–415. doi: 10.1109/LGRS.2019.2924266
|
[71] |
田壮壮, 占荣辉, 胡杰民, 等. 基于卷积神经网络的SAR图像目标识别研究[J]. 雷达学报, 2016, 5(3): 320–325. doi: 10.12000/JR16037
TIAN Zhuangzhuang, ZHAN Ronghui, HU Jiemin, et al. SAR ATR based on convolutional neural network[J]. Journal of Radars, 2016, 5(3): 320–325. doi: 10.12000/JR16037
|
[72] |
王俊, 郑彤, 雷鹏, 等. 深度学习在雷达中的研究综述[J]. 雷达学报, 2018, 7(4): 395–411. doi: 10.12000/JR18040
WANG Jun, ZHENG Tong, LEI Peng, et al. Study on deep learning in radar[J]. Journal of Radars, 2018, 7(4): 395–411. doi: 10.12000/JR18040
|
[73] |
苏宁远, 陈小龙, 关键, 等. 基于卷积神经网络的海上微动目标检测与分类方法[J]. 雷达学报, 2018, 7(5): 565–574. doi: 10.12000/JR18077
SU Ningyuan, CHEN Xiaolong, GUAN Jian, et al. Detection and classification of maritime target with micro-motion based on CNNs[J]. Journal of Radars, 2018, 7(5): 565–574. doi: 10.12000/JR18077
|
[74] |
KIM B K, KANG H S, and PARK S O. Drone classification using convolutional neural networks with merged Doppler images[J]. IEEE Geoscience and Remote Sensing Letters, 2017, 14(1): 38–42. doi: 10.1109/LGRS.2016.2624820
|
[75] |
MENDIS G J, WEI Jin, and MADANAYAKE A. Deep learning cognitive radar for micro UAS detection and classification[C]. 2017 Cognitive Communications for Aerospace Applications Workshop, Cleveland, USA, 2017: 1–5. doi: 10.1109/CCAAW.2017.8001610.
|
[76] |
苏宁远, 陈小龙, 陈宝欣, 等. 雷达海上目标双通道卷积神经网络特征融合智能检测方法[J]. 现代雷达, 2019, 41(10): 47–52, 57. doi: 10.16592/j.cnki.1004-7859.2019.10.009
SU Ningyuan, CHEN Xiaolong, CHEN Baoxin, et al. Dual-channel convolutional neural networks feature fusion method for radar maritime target intelligent detection[J].Modern Radar, 2019, 41(10): 47–52, 57. doi: 10.16592/j.cnki.1004-7859.2019.10.009
|
[77] |
陈唯实, 刘佳, 陈小龙, 等. 基于运动模型的低空非合作无人机目标识别[J]. 北京航空航天大学学报, 2019, 45(4): 687–694. doi: 10.13700/j.bh.1001-5965.2018.0447
CHEN Weishi, LIU Jia, CHEN Xiaolong, et al. Non-cooperative UAV target recognition in low-altitude airspace based on motion model[J]. Journal of Beijing University of Aeronautics and Astronautics, 2019, 45(4): 687–694. doi: 10.13700/j.bh.1001-5965.2018.0447
|
[78] |
陈唯实, 万健, 李敬. 基于机场探鸟雷达数据的鸟击风险评估[J]. 北京航空航天大学学报, 2013, 39(11): 1431–1436.
CHEN Weishi, WAN Jian, and LI Jing. Bird strike risk assessment with airport avian radar data[J]. Journal of Beijing University of Aeronautics and Astronautics, 2013, 39(11): 1431–1436.
|
[79] |
陈唯实, 张洁, 卢贤锋. 基于探鸟雷达数据的机场鸟情分析[J]. 民用航空, 2020, (1): 43–45.
CHEN Weishi, ZHANG Jie, and LU Xianfeng. Airport bird situation analysis based on avian radar data[J]. China Civil Aviation, 2020, (1): 43–45.
|
[80] |
KIM B K, KANG H S, and PARK S O. Experimental analysis of small drone polarimetry based on micro-Doppler signature[J]. IEEE Geoscience and Remote Sensing Letters, 2017, 14(10): 1670–1674. doi: 10.1109/LGRS.2017.2727824
|
[81] |
章鹏飞, 李刚, 霍超颖, 等. 基于双雷达微动特征融合的无人机分类识别[J]. 雷达学报, 2018, 7(5): 557–564. doi: 10.12000/JR18061
ZHANG Pengfei, LI Gang, HUO Chaoying, et al. Classification of drones based on micro-Doppler radar signatures using dual radar sensors[J]. Journal of Radars, 2018, 7(5): 557–564. doi: 10.12000/JR18061
|
[82] |
罗迎, 倪嘉成, 张群. 基于“数据驱动+智能学习”的合成孔径雷达学习成像[J]. 雷达学报, 2020, 9(1): 107–122. doi: 10.12000/JR19103
LUO Ying, NI Jiacheng, and ZHANG Qun. Synthetic aperture radar learning-imaging method based on data-driven technique and artificial intelligence[J]. Journal of Radars, 2020, 9(1): 107–122. doi: 10.12000/JR19103
|
[83] |
牟效乾, 陈小龙, 苏宁远, 等. 基于时频图深度学习的雷达动目标检测与分类[J]. 太赫兹科学与电子信息学报, 2019, 17(1): 105–111. doi: 10.11805/TKYDA201901.0105
MOU Xiaoqian, CHEN Xiaolong, SU Ningyuan, et al. Radar detection and classification of moving target using deep convolutional neural networks on time-frequency graphs[J]. Journal of Terahertz Science and Electronic Information Technology, 2019, 17(1): 105–111. doi: 10.11805/TKYDA201901.0105
|
[84] |
SUN Hongbo, OH B S, GUO Xin, et al. Improving the Doppler resolution of ground-based surveillance radar for drone detection[J]. IEEE Transactions on Aerospace and Electronic Systems, 2019, 55(6): 3667–3673. doi: 10.1109/TAES.2019.2895585
|
[85] |
许稼, 彭应宁, 夏香根, 等. 空时频检测前聚焦雷达信号处理方法[J]. 雷达学报, 2014, 3(2): 129–141. doi: 10.3724/SP.J.1300.2014.14023
XU Jia, PENG Yingning, XIA Xianggen, et al. Radar signal processing method of space-time-frequency focus-before-detects[J]. Journal of Radars, 2014, 3(2): 129–141. doi: 10.3724/SP.J.1300.2014.14023
|
[86] |
陈曾平, 张月, 鲍庆龙. 数字阵列雷达及其关键技术进展[J]. 国防科技大学学报, 2010, 32(6): 1–7. doi: 10.3969/j.issn.1001-2486.2010.06.001
CHEN Zengping, ZHANG Yue, and BAO Qinglong. Advance in digital array radar and its key technologies[J]. Journal of National University of Defense Technology, 2010, 32(6): 1–7. doi: 10.3969/j.issn.1001-2486.2010.06.001
|
[87] |
CHEN Xiaolong, GUAN Jian, LI Xiuyou, et al. Effective coherent integration method for marine target with micromotion via phase differentiation and radon-Lv’s distribution[J]. IET Radar,Sonar&Navigation, 2015, 9(9): 1284–1295. doi: 10.1049/iet-rsn.2015.0100
|
[88] |
KLARE J, BIALLAWONS O, and CERUTTI-MAORI D. UAV detection with MIMO radar[C]. The 2017 18th International Radar Symposium, Prague, Czech Republic, 2017. doi: 10.23919/IRS.2017.8008140.
|
1. | 汪思源,曲毅,陈怡君. 基于U-Net的涡旋电磁波雷达成像方法. 空军工程大学学报. 2024(03): 77-85 . ![]() | |
2. | 潘浩然,马晖,胡敦法,刘宏伟. 基于涡旋电磁波新体制的雷达前视三维成像. 雷达学报. 2024(05): 1109-1122 . ![]() | |
3. | 毛德庆,杨建宇,杨明杰,张永超,张寅,黄钰林. IAA-Net:一种实孔径扫描雷达迭代自适应角超分辨成像方法. 雷达学报. 2024(05): 1073-1091 . ![]() | |
4. | 马晖,胡敦法,师竹雨,刘宏伟. 基于涡旋电磁波的雷达应用研究进展. 现代雷达. 2023(05): 27-41 . ![]() | |
5. | 袁航,罗迎,陈怡君,苏令华. 基于反正弦圆环天线阵列的二维成像. 北京航空航天大学学报. 2023(06): 1487-1494 . ![]() |
日期(2016年) | 垂直有效波数(rad/m) | 时间基线(天) | 距离向/方位向分辨率(m) | 中心入射角 (°) | 极化方式 |
0616—0630 (BL1) | 0.013~0.015 | ||||
0630—0714 (BL2) | 0.010~0.011 | 14 | 2.86/2.97 | 38.99 | Full |
0811—0825 (BL3) | 0.009~0.010 |
模型参数 | BL1 | BL2 | BL3 |
Sscene | 0.69 | 0.78 | 0.78 |
Cscene | 9.88 | 10.08 | 11.14 |
林分样地编号 | BL1 P值 / 森林高度(m) | BL2 P值 / 森林高度(m) | BL3 P值 / 森林高度(m) | 多基线融合结果(m) | 实测森林高度(m) |
1 | 0.130 / 17.82 | 0.113 / 17.02 | 0.081 / 16.89 | 17.82 | 14.43 |
2 | 0.116 / 14.38 | 0.104 / 15.30 | 0.091 / 16.52 | 14.38 | 14.20 |
3 | 0.092 / 12.46 | 0.075 / 15.83 | 0.135 / 11.34 | 11.34 | 9.80 |
4 | 0.103 / 15.21 | 0.111 / 15.34 | 0.119 / 14.19 | 14.19 | 16.00 |
5 | 0.106 / 6.86 | 0.106 / 7.24 | 0.131 / 8.31 | 8.31 | 10.70 |
6 | 0.110 / 12.98 | 0.083 / 14.67 | 0.118 / 11.89 | 11.89 | 13.50 |
7 | 0.114 / 13.35 | 0.096 / 15.30 | 0.101 / 16.10 | 13.35 | 13.43 |
8 | 0.079 / 14.29 | 0.106 / 16.15 | 0.117 / 16.22 | 16.22 | 16.95 |
9 | 0.069 / 12.12 | 0.090 / 17.63 | 0.060 / 12.30 | 17.63 | 20.10 |
10 | 0.104 / 12.33 | 0.089 / 13.67 | 0.102 / 11.72 | 12.33 | 15.60 |
11 | 0.075 / 18.34 | 0.103 / 16.75 | 0.154 / 10.16 | 10.16 | 13.30 |
12 | 0.113 / 9.08 | 0.134 / 9.46 | 0.106 / 12.69 | 9.46 | 11.00 |
13 | 0.086 / 13.76 | 0.096 / 9.07 | 0.109 / 16.00 | 16.00 | 16.40 |
14 | 0.197 / 10.17 | 0.230 / 8.71 | 0.186 / 9.51 | 8.71 | 6.00 |
15 | 0.103 / 14.59 | 0.064 / 19.17 | 0.128 / 15.40 | 15.40 | 14.70 |
日期(2016年) | 垂直有效波数(rad/m) | 时间基线(天) | 距离向/方位向分辨率(m) | 中心入射角 (°) | 极化方式 |
0616—0630 (BL1) | 0.013~0.015 | ||||
0630—0714 (BL2) | 0.010~0.011 | 14 | 2.86/2.97 | 38.99 | Full |
0811—0825 (BL3) | 0.009~0.010 |
模型参数 | BL1 | BL2 | BL3 |
Sscene | 0.69 | 0.78 | 0.78 |
Cscene | 9.88 | 10.08 | 11.14 |
林分样地编号 | BL1 P值 / 森林高度(m) | BL2 P值 / 森林高度(m) | BL3 P值 / 森林高度(m) | 多基线融合结果(m) | 实测森林高度(m) |
1 | 0.130 / 17.82 | 0.113 / 17.02 | 0.081 / 16.89 | 17.82 | 14.43 |
2 | 0.116 / 14.38 | 0.104 / 15.30 | 0.091 / 16.52 | 14.38 | 14.20 |
3 | 0.092 / 12.46 | 0.075 / 15.83 | 0.135 / 11.34 | 11.34 | 9.80 |
4 | 0.103 / 15.21 | 0.111 / 15.34 | 0.119 / 14.19 | 14.19 | 16.00 |
5 | 0.106 / 6.86 | 0.106 / 7.24 | 0.131 / 8.31 | 8.31 | 10.70 |
6 | 0.110 / 12.98 | 0.083 / 14.67 | 0.118 / 11.89 | 11.89 | 13.50 |
7 | 0.114 / 13.35 | 0.096 / 15.30 | 0.101 / 16.10 | 13.35 | 13.43 |
8 | 0.079 / 14.29 | 0.106 / 16.15 | 0.117 / 16.22 | 16.22 | 16.95 |
9 | 0.069 / 12.12 | 0.090 / 17.63 | 0.060 / 12.30 | 17.63 | 20.10 |
10 | 0.104 / 12.33 | 0.089 / 13.67 | 0.102 / 11.72 | 12.33 | 15.60 |
11 | 0.075 / 18.34 | 0.103 / 16.75 | 0.154 / 10.16 | 10.16 | 13.30 |
12 | 0.113 / 9.08 | 0.134 / 9.46 | 0.106 / 12.69 | 9.46 | 11.00 |
13 | 0.086 / 13.76 | 0.096 / 9.07 | 0.109 / 16.00 | 16.00 | 16.40 |
14 | 0.197 / 10.17 | 0.230 / 8.71 | 0.186 / 9.51 | 8.71 | 6.00 |
15 | 0.103 / 14.59 | 0.064 / 19.17 | 0.128 / 15.40 | 15.40 | 14.70 |