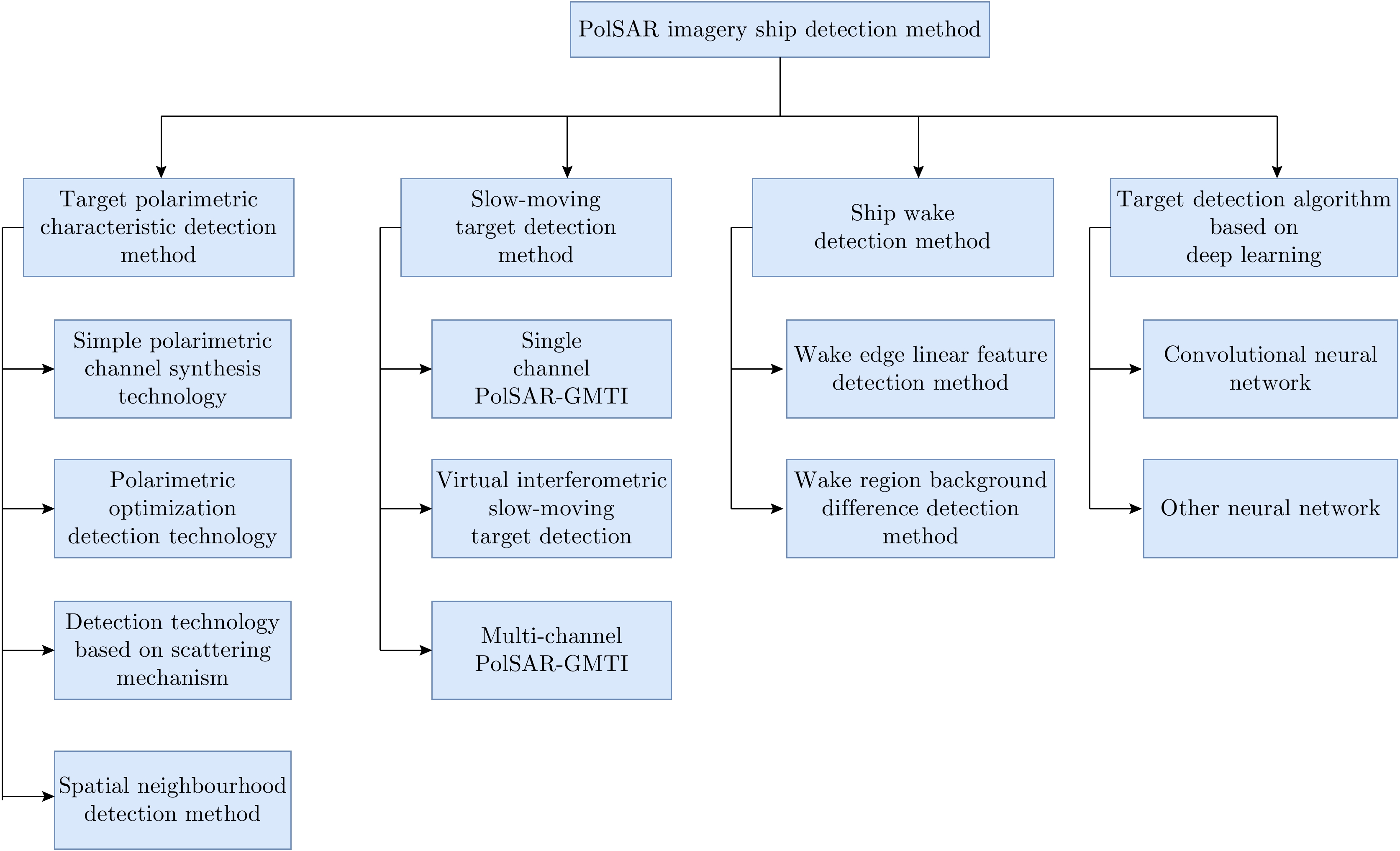
Citation: | LIU Tao, YANG Ziyuan, JIANG Yanni, et al. Review of ship detection in polarimetric synthetic aperture imagery[J].Journal of Radars, 2021, 10(1): 1–19. doi: 10.12000/JR20155 |
Synthetic Aperture Radar (SAR) is an active microwave remote sensing device. SAR uses virtual array and pulse compression technologies to obtain two-dimensional high-resolution images of ground objects. Polarization is an important attribute of electromagnetic waves, and the polarization characteristics of a target are one of its important inherent attributes, which can be used in target detection and recognition. Polarimetric SAR (PolSAR) is generated by inputting polarimetric information into the SAR system. The PolSAR system, which has retained complete target electromagnetic scattering characteristics, has become an important tool of modern radar imaging technology, and it has been widely used in military reconnaissance terrain mapping, environmental and natural disaster monitoring, ship detection, and other fields[1]. In the past 30 years, many scholars have performed considerable work in the field of polarimetric radar remote sensing, which has laid a solid foundation for the application of polarimetric radar remote sensing and made significant achievements. Notably, Boerner and his international collaborators have made remarkable contributions. He is not only an active promoter of the polarimetric radar theory and its application but also an active disseminator of the polarimetric radar theory. The first practical orthogonal PolSAR system was Airborne SAR (AIRSAR) developed by the National Aeronautics and Space Administration Jet Propulsion Laboratory (NASA JPL)[2] in 1985 based on time-sharing polarimetric measurement technology. The successful acquisition of data has stimulated the analysis and application of PolSAR data, and since then, the PolSAR system has entered a period of rapid development. Thus far, the typical PolSAR systems at home and abroad mainly include[3]: (1) Airborne SAR: USA’s AIRSAR system, Australia’s AuSAR system, Denmark’s EMISAR system, New Zealand’s PHARUS system, Japan’s PISAR system, Canada’s CV-580 system, Germany’s DOSAR MEMPHIS ESAR system, and France’s STORM system and (2) Spaceborne SAR: Japan’s ALOS/PALSAR system, Germany’s Terrasar-X system, and Canada’s RADARSAT-1/2 system. On August 10, 2016, China’s first civilian C-band PolSAR satellite, Gaofen-3 (GF-3), was successfully launched from the Taiyuan Satellite Launch Center, reaching the advanced international level. GF-3 has been widely used in marine disaster reduction, water conservation, meteorology, and other fields.
With the rapid development of the PolSAR system, PolSAR image interpretation technology has been the focus of considerable attention. Target detection methods based on PolSAR images have become a frontier topic in the field of SAR information processing. Many research institutions at home and abroad have conducted fruitful work in the field of PolSAR target detection. Lincoln Laboratory, USA; Canada Centre for Remote Sensing, Canada; University of Niigata, Japan; German Aerospace Center (DLR), Germany; Defence Science and Technology Organisation (DSTO), Australia; Stirling University, UK; Trumsø University, Norway; Chinese Academy of Sciences, China; Tsinghua University, China; National University of Defense Technology, China; University of Electronic Science and Technology, China; Xi’an University of Electronic Science and Technology, China; Naval University of Engineering, China; and other research institutions have made outstanding contributions[3]. As the representative research institutions, NASA JPL and Lincoln Laboratory have conducted pioneering works on PolSAR image target detection, classification, and recognition and obtained a large number of experimental and theoretical results. Research results show that polarimetric information will further improve the radar target detection, classification, and recognition performance[4]. Novak of Lincoln Laboratory has made a fundamental contribution to the field of target polarimetric characteristic detection. The most common method of ship target detection in PolSAR image is Constant False Alarm Rate (CFAR) because the ship target has a stronger scattering echo than the sea clutter. In the case that the prior information of the target is unknown, the CFAR detection method can achieve a better performance than other detection methods. However, with the diversity of radar platform parameters and the complexity of sea surface environments, addressing the problems associated with sea clutter modeling and parameter estimation, slow and small target detection, dense target detection, and other issues remain a challenge in PolSAR ship detection.
By summarizing the well-known PolSAR ship detection algorithms, this paper provides the development history and future trend of PolSAR ship detection and some valuable suggestions for subsequent research. The framework of this overview is shown in Fig. 1. At present, PolSAR ship detection can be divided into four categories, i.e., (1) target polarimetric characteristic detection method, (2) slow-moving target detection method, (3) ship wake detection method, and (4) target detection algorithm based on deep learning. The target polarimetric characteristic detection method is mainly applied to ship detection when there is a certain difference between polarimetric characteristics of ship and clutter. The slow-moving target detection method is mainly applied to the situation in which the polarimetric characteristic difference between the target and clutter is small, but there is a certain velocity difference between the target and clutter. Meanwhile, the target motion information can be extracted to obtain the real-time sea surface situation. The ship wake detection method is not used to detect the ship target itself but to detect the wake generated by ship motion, which is mainly used for small and stealth target detection. The data-driven deep learning method is an important tool of Artificial Intelligence (AI) and does not require manual target feature extraction. This method has achieved great success in the intelligent interpretation of PolSAR images. CFAR detection is essentially statistical signal processing, which can be regarded as the follow-up process of the aforementioned methods. Thus, a series relationship is observed between the CFAR detection method and the aforementioned methods. LIU et al.[5] have done considerable research on this, and only a representative introduction is listed in this paper.
The most common method of PolSAR image detection is based on the statistical characteristics of SAR pixels[6]. To utilize the statistical similarity and spatial correlation of pixels, the pixel block detection method was developed[7]. PolSAR ship characteristic detection methods can be divided into two categories, i.e., pixel level and pixel block[7]. Pixel level detection methods mainly include simple polarimetric channel synthesis technology, polarimetric optimization detection technology, and detection technology based on the scattering mechanism[8]. Pixel block detection methods, also known as spatial neighborhood detection methods, mainly include superpixel and neighborhood matrix detection algorithms.
As the number of polarimetric channels increased, simple polarimetric channel synthesis techniques have emerged[9]. Simple polarimetric channel synthesis has two schemes. The first scheme is to detect and process each polarimetric channel separately and to fuse the final detection results, which is called decision-level fusion, using a Single Channel Detector (SCD)[9]. The second scheme, which is based on pixels, is to synthesize the data of different polarimetric channels and perform CFAR detection on the synthesized images in a single-channel manner, which is called signal-level fusion and can be regarded as the detection quantity obtained by the linear weighting of the scattering vector[10] using a Span Detector (SD) and Optimal Span Detector (OSD)[10]. A large number of experimental results show that the detection performance of polarimetric channel synthesis is better than that of single-polarization SAR[10]. This method is relatively simple; however, it is no longer used, except for simple scenarios and situations with low performance requirements.
Polarimetric optimization refers to the information processing technology of extracting object characteristics from PolSAR images by comprehensively utilizing the information of different polarimetric channels according to a certain optimization objective function. Polarimetric optimization is an upgrade of simple polarimetric channel synthesis. In 1988, Boerner[11] proposed the Polarimetric Matched Filter (PMF) based on the maximum Signal-to-Noise Ratio (SNR) criterion. The PMF is an optimal linear filter, which can suppress clutter and improve the signal-to-clutter ratio (SCR) by weighting the polarimetric scattering vector[11]. In 1989, Novak et al.[12] proposed an Optimal Polarimetric Detector (OPD) based on the principle of the likelihood ratio test. The proposed method reaches the theoretical upper limit of the polarization detector (with the prior information of the target required). The object covariance matrix in the OPD is replaced by the product of the unit matrix and the polarimetric channel power, and the identity likelihood ratio test detector can be obtained[13]. In 1990, Novak and Burl[14] proposed a Polarimetric Whitening Filter (PWF) to compensate for the defect that the OPD requires prior information by taking the clutter fluctuation as the optimization objective. YANG et al.[15] proposed the Optimization of Polarimetric Contrast Enhancement (OPCE) based on the maximum SNR criterion by optimizing the polarimetric mode of transmitting and receiving and achieved a detection effect that is close to the matched filter. YANG et al.[16] proposed a method based on the Minimal Clutter-to-Signal Ratio (MCSR) to enhance the polarimetric contrast and concluded that the PMF was a special case of the MCSR. The MCSR method has a good target detection performance in a specific environment; however, it cannot identify the optimal detection dimension of the subspace. Novak[17] noted that detection performance depends not only on SNR but also on clutter fluctuation. The performance comparison of polarimetric optimization detection technology is shown in Fig. 2. The experimental results show that the OPD generally has the best performance, and the OPCE is generally less effective than the PWF[18].
To extend the work of Novak et al. to the multi-view situation, Lopes and LIU[19,20] extended the PWF to the multi-look PWF. Based on PWF statistical modeling and parameter estimation, LIU et al.[21] conducted in-depth research on the CFAR characteristics of PolSAR ship detection. According to the sea clutter under different conditions, the CFAR analytical expressions of different statistical distributions are deduced, and the ideal CFAR results are obtained. To some extent, the problems of CFAR statistical modeling and parameter estimation under the influence of varying radar platform parameters and complex sea surface environments are solved. Fig. 3 shows the PWF-based CFAR detection results with clutter background characterized by the IBeta distribution product model. Notably, when the statistical model is consistent with the measured data, CFAR has the best performance, whereas other statistical models (e.g., K-Wishart, G0-Wishart, and lognormal models) have many false alarms. Inspired by the aforementioned works, GAO et al.[22–25] also investigated the statistical modeling and parameter estimation of single PolSAR images.
For a dense target environment, TAO[27] proposed an adaptive truncation method to estimate the statistical model parameters of SAR images and achieved ideal detection results. LIU[28] extended the method to PolSAR images, deduced the probability density function of the adaptive truncated polarimetric covariance matrix, and achieved ideal results in the dense target environment. The PWF detection results on the GF-3 image are shown in Fig. 4, where Fig. 4(a)–Fig. 4(e) represent the different parameter estimation methods and (f) represent the Pauli image of the RADARSAT-2 data, with the yellow rectangle denoting the false alarm and the red rectangle denoting the missing alarm. Notably, the effect of Fig. 4(a) on the accuracy of parameter estimation after truncation is significantly improved, which also indicates the necessity of accurate parameter estimation for clutter in dense areas.
At present, the polarimetric optimization method is gradually combined with the interpretable machine learning method, and target detection is conducted by adopting classification optimization criteria[29], which is a new method to improve the detection performance of small targets and can be used as the development direction of subsequent polarization optimization detection technology.
Given the different scattering mechanisms between ship target and ocean surface, the difference between polarimetric characteristics can distinguish the ship from the clutter. Thus, the detection method based on the polarimetric scattering characteristics has strong physical interpretability. Because sea clutter has reflection symmetry, Nunziata et al.[30] proposed a Reflection Symmetry (RS) detector, which uses the correlation between co-polarimetric and cross-polarimetric channels to detect targets. WANG et al.[31] conducted a further theoretical analysis of the RS detector and deduced the CFAR analytical expression and parameter estimation method of the detector. From the perspective of comparing the polarimetric scattering energy of clutter and ship target, Marino et al.[32,33] deduced the Polarimetric Notch Filter (PNF) based on geometric disturbance and obtained the corresponding analytical expression of statistical distribution[33]. Gao et al.[34–36] applied the PNF to high sea state conditions and mixed polarimetric and interferometric conditions and achieved ideal results. In 2020, LIU et al.[8] modified the PNF expression, called it the new PNF (NPNF), considering the real physical significance of energy in electromagnetic scattering, and achieved a better detection performance than the original PNF. The experimental comparison results are shown in Fig. 5(a). The effect of NPNF is similar to that of PWF. The comparison of the detection effects of PNF and NPNF is shown in Fig. 5(b).
Another detection method based on the scattering mechanism is to use the polarimetric decomposition theory. Polarimetric decomposition is an effective tool to analyze the scattering mechanism of targets. Polarimetric decomposition can decompose the scattering or covariance matrix according to different physical scattering types and can be effectively applied to ship target detection in PolSAR images[37]. In 1999, Cameron decomposition was first applied to SIR-C data from spaceborne imaging radar for wide-area surface ship target detection by Ringrose and Harris[38]. In 2000, based on the Cloude-Pottier decomposition, Touzi et al.[39] introduced the detection amount of polarimetric entropy derived from the polarimetric covariance matrix to detect the target through the eigenvalue of polarimetric entropy and scattering angle, which effectively improved the difference between the target and the background and achieved a better ship detection effect[40]. In 2009, CHEN et al.[41] proposed the concept of polarimetric cross-entropy and proved the effectiveness of feature detection using AIRSAR data. In 2013, Sugimoto et al.[42] applied the decomposition of the four-component model proposed by Yamaguchi to ship target detection using ALOS PALSAR measured data. The advantage of this algorithm lies in the simplicity of interpretation and execution. YANG et al.[43,44] added the scattering mechanism to OPCE and proposed a Generalized OPCE (GOPCE) method. Their results show that the addition of scattering similarity significantly improves the target detection capability.
In 2015, Touzi et al.[45] used the optimal characteristics of depolarization to detect ships and proved that the algorithm could effectively detect ship targets using the RADARSAT-2 measured data. In 2018, GAO et al.[36] combined polarimetric entropy and scattering energy to detect ships in PolSAR images and achieved good results, as shown in Fig. 6. This method reflects the advantages of the fusion of energy and polarimetric domain features and is more conducive to target detection. In 2019, Bordbari et al.[46] divided the scattering mechanism into two categories, i.e., target scattering mechanism for the target and nontarget scattering mechanism for the clutter, and adopted the subspace projection method to suppress clutter and significantly improve the target detection performance. At present, a single polarimetric decomposition model cannot easily and effectively express the difference between target and clutter. Thus, new polarimetric decomposition models are investigated, and different component coefficients of different polarimetric decomposition models are reconstructed into new feature vectors. Moreover, the Fisher classification criterion or other feature vector space can improve the detection performance[47], which is also the trend of ship target detection based on the polarimetric decomposition theory.
Traditional PolSAR imagery processing is mostly based on a single pixel but ignores the relationship between neighborhood pixels. Therefore, its performance will be reduced when the clutter scattering characteristics are similar to the ships’ characteristics, such as ship detection in ports. To utilize the correlation between pixel and its neighborhood, the pixel block detection method has been developed. Recent studies show that the fusion of spatial and polarimetric information can considerably enhance the target detection capability, and the target detection effect can be further enhanced by extracting the spatial information[6,48]. At present, detection based on superpixel and neighborhood matrices is mainly used[7,48].
The essence of a superpixel is a set of points with similar scattering characteristics; thus, the definition of similarity is its core. WANG et al.[49] proposed three superpixel-level similarity definitions to enhance the SCR and built an automatic detection algorithm based on this. Finally, using the RADARSAT-2 data, even at a low Signal Clutter Ratio (SCR), the method can still achieve a low Probability of False Alarm (PFA). Another study[50] proposed a similar superpixel segmentation method based on the polarimetric scattering similarity of ship targets and sea clutter. CUI et al.[51] determined the Similar Pixel Number (SPN) of PolSAR images by conducting the similarity test, and a target detection method based on this feature is proposed. The RADARSAT-2 and GF-3 measured data show that the introduction of airspace and polarimetric information can considerably enhance the detection capability of ship targets in PolSAR images. As shown in Fig. 7, the green rectangle denotes correct detection, the yellow rectangle denotes false alarm, and the red rectangle denotes missing alarm. Fig. 7(a)–Fig. 7(d) represent the different detection methods. The results show that the SPN method has obvious advantages.
Huang et al.[52] proposed a PolSAR image significance detection model based on the distance between blocks. The polarimetric coherence matrix was used to measure the similarity between polarimetric pixel blocks at both local and global scales. Jäger et al.[53] defined PolSAR image entropy according to polarimetric and spatial information and proposed a method to detect PolSAR image salient regions. Wang et al.[54] proposed an image salient region detection method based on the similarity test of the pixel block polarimetric covariance matrix. LIN et al.[55] first proposed the method of ship detection in single PolSAR images using the superpixel-level Fisher vector. Then, in their doctoral dissertation, they compared PolSAR images with RGB images and extended the edge sensing superpixel segmentation method to make the superpixel ship detection method applicable to PolSAR images.
To construct the neighborhood matrix, ZHANG et al.[56,57] drew on the concept of optical local binary coding and proposed a polarimetric covariance matrix and a complete polarimetric covariance difference matrix using the amplitude, phase, and spatial information to distinguish ship target pixel and sea clutter pixel. However, the final matrix form lacks some good properties, such as it is not a positive semidefinite matrix and it is difficult to interpret as a practical target. Ships and clutter are both spatially correlated, and ships will change the spatial correlation of surrounding clutter. Sidelobes also affect the correlation of neighborhood pixels. Therefore, LIU et al.[7] proposed the Neighborhood Polarimetric Covariance Matrix (NPCM) and a detection method based on the NPCM. Compared with the results of existing methods, their results show that, at present, the new method has outstanding performance and is nearly the best polarimetric detector, as shown in Fig. 8.
The NPCM combines polarimetric and spatial characteristics and significantly increases the dissociability between ships and sea clutter. However, the best dimension of the neighborhood spatial correlation matrix is difficult to determine and the total dimension is high. In the future, the development direction of the neighborhood matrix is to reduce its dimension to avoid dimensional disaster while maintaining the detection performance. Simultaneously, the neighborhood matrix information can be combined with the superpixel segmentation, which is expected to improve the spatial detection performance.
With the continuous deterioration of the sea surface environments, the detection of the slow, small ship submerged in the sea clutter becomes more difficult. Ground Moving Target Indication (GMTI) has become one of the important functions that the PolSAR system needs to complete to more comprehensively grasp the situation of the naval battlefield and obtain the target motion information. Moving target detection algorithms are divided into two categories, i.e., range-Doppler detection algorithms and image detection algorithms[58]. Compared with that in the range-Doppler domain, the operation in the SAR image domain has the advantages of low design requirements, small computation, and easy implementation. Thus, this paper focuses on detection in the image domain. GAO et al.[59–61] conducted in-depth research on slow-moving target detection on the image domain in a single polarimetric situation. Here we mainly focus on the utilization of polarimetric information. Thus, the PolSAR-GMTI system is divided into single-channel PolSAR-GMTI and multichannel PolSAR-GMTI, where a single channel refers to a single radar and a multichannel refers to two or more radars.
In 1995, Park et al.[62] conducted in-depth research on the polarimetric Space-Time Adaptive Processing (STAP) algorithm and proposed the joint processing method of space-time-polarization adaptive signal. Their research results show that the addition of polarimetric information can improve the moving target detection performance. In 2003, Park et al.[63] further analyzed the potential of polarimetric information to improve the performance of the STAP algorithm. Wang et al.[64] proposed a moving target detection algorithm based on polarimetric-interferometric optimization technology. On this basis, Friedlander et al.[65] used the VSAR velocity estimation principle to estimate the moving target velocity based on the results after polarimetric-interferometric optimization. LIU et al.[66] from NUDT analyzed the coherence of frequency domain residual interference in PolSAR images. For moving targets located on random rough surfaces, such as highways, Yeremy et al. in Canada, funded by the Ministry of National Defence of Canada, conducted a full PolSAR-GMTI test in Petawawa on June 8, 2002, and in Ottawa on September 24, 2002, using the CV-580 system and obtained a large amount of data. Using these data, Matter et al.[67] published a special AD report in 2005 and investigated the feasibility of using PolSAR data for moving target detection. Two PolSAR moving target detection algorithms are mentioned in the report. One is based on single PolSAR data, and the other is the processing method of Along-track Interferometry (ATI) based on two cross-polarimetric channels. The cross-polarimetric ATI method uses the reciprocity of cross-polarimetric images of stationary targets through ATI processing of SAR images of two cross-polarimetric channels[67] to achieve moving target detection. Of course, the cross-polarimetric ATI method can also use two cross-polarimetric channel SAR images for the Displaced Phase Center Antenna (DPCA) method to achieve moving object detection[67]. ZOU et al.[68] proposed an algorithm combining the PWF and time–frequency analysis method. This method enhanced the SNR of the moving target by utilizing polarimetric information, enhanced the visibility of the target image, and completed the detection of the linear contour of the moving target image by Radon transform.
LIU et al.[69,70] analyzed the influence of motion on PolSAR images. Their results show that the phase difference between HV and VH images is 0 for the scatterer with reciprocity, whereas the phase difference between HV and VH sidelobes is
Multichannel SAR increases the spatial information and can use both spatial and temporal information simultaneously, which can suppress the stationary clutter, detect the moving target more effectively, and solve the inherent blurring problem. Polarimetric information helps improve the interferometric quality, estimation accuracy of the interferometric phase[58], and detection performance of slow-moving targets[71]. To overcome the low performance limit of the Minimum Detectable Velocity (MDV) for single-platform PolSAR-GMTI, which is limited by size, LI et al.[72] proposed the use of the virtual baseline in the mode of alternating transmitting and receiving by the fully polarimetric radar and combined three new virtual baselines to obtain a better MDV. Based on considerable research on single-polarimetric multichannel SAR-GMTI, Defence Research and Development Canada designed a dual-channel polarimetric beam mode for RS-2, which combines PolSAR imaging with GMTI functions using dual-channel and time-sharing polarimetric techniques. Chiu et al.[73] proved that this method helps detect ship targets in the Arctic glacier region. Given that the RADARSAT Constellation Mission (RCM), which was launched in 2019, does not have a dedicated GMTI function, Chiu et al.[74] proposed the moving target detection method of double PolSAR images based on cross-polarimetric information to generate shadows, thereby providing an auxiliary GMTI function.
Australian scholars Stacy and Preiss[71] extended ATI amplitude-phase detection to the GLRT method similar to the single-channel situation but did not conduct in-depth formula derivation and analysis. To address the difficulties of GMTI in the current interferometric SAR (InSAR), Zhang Peng et al.[75,76] used Polarimetric ATI SAR (PolATInSAR) to improve the detection performance of slow-moving targets. Their results show that the new method can significantly improve the detection probability and radial velocity estimation accuracy of the interference system for slow and small ship targets and can easily achieve CFAR detection. The simulation results are shown in Fig. 10. With the successful launch of the RCM and the subsequent development of China’s GF series constellation architecture, the need for multi-station polarimetric-interferometric processing has become more obvious.
The current PolATInSAR studies lack the acquisition of measured data. To facilitate research on PolATInSAR and put it into practice as soon as possible, the subaperture decomposition method can be adopted to obtain the virtual interferometric effect. In 1985, Japanese scientist Ouchi[77] first analyzed the characteristics of moving targets in multi-look sub-images in the time domain and indicated that there is a certain time delay between synthetic aperture centers of SAR subaperture images and the two-dimensional velocity of moving targets will produce imaging differences between multi-view image sequences. Based on Ouchi’s research, in 1996, German scientist Martin Kirscht[78] first applied the multi-view processing method to SAR-GMTI and proposed the single-look image sequence method, which is also known as the subaperture decomposition method. This method achieves the goal of canceling clutter and retaining the moving target by subtracting multiple subaperture image sequences. Scholars from the Chinese Academy of Sciences and Beijing Aerospace University[79–82] have made many improvements to the subaperture decomposition method and combined it with the multichannel SAR-GMTI method to achieve good results. In 2015, Marino et al.[83] derived the target detection algorithm after subaperture decomposition and summarized the results of detection performance comparison. In the future, subaperture decomposition will be extended to the PolSAR direction by the dimension extension method to obtain a better PolSAR-GMTI effect.
Wake detection, which has an extensive development space, can acquire the course and speed of ship targets and other information and provide a new idea for the detection of PolSAR small targets and stealth targets. Wake detection can be summed up by the difference in energy between the wake and the background. Wake detection is mainly based on the linear feature extraction of the wake edge and the difference between the wake region and the background scattering characteristics. CHONG et al.[84] considered the following issues: (1) wake is a linear feature body with a certain width; (2) the wake may be lighter or darker than the sea background; (3) wakes are not necessarily straight, but sometimes curved; (4) SAR images have inherent speckle noise; and (5) other non-wake linear structures can be detected in the image.
In 1992, Schuler et al.[85] investigated the polarimetric characteristics of one arm of the P-band Kelvin wake. In 1999, Pottier et al.[86] proposed the classification method of ship wakes using the feature vectors in the polarimetric eigenvalue decomposition. This method uses a neural network to classify the simulated fully polarimetric Kelvin wake data, and the classified samples are composed of the rotation invariants after polarimetric decomposition. However, the classification results do not specify the physical meaning of the wakes represented by these features, nor do they verify the measured data[87]. In 1999, Hennings et al.[88] analyzed the Kelvin arm model in SAR images and concluded that the visibility of the Kelvin arm on radar images largely depends on the radar polarization mode rather than the radar frequency. In HH polarization, the visibility of the Kelvin arm on radar images is higher than that of VV polarization. When the incident angle is small, the Kelvin arm has high visibility on radar images[89]. In 2001, Imbo et al.[87,90] investigated wake features in PolSAR images and conducted a Radon transform of the polarimetric covariance matrix to detect ship wakes. Two methods of wake type detection are discussed in this paper. One is to use the optimal channel combination after PWF processing for wake detection, and the other is to perform Radon transform in the six channels of the polarimetric covariance matrix, use the Mahalanobis distance to judge the peak value, and use the inverse transform method to detect the wake. The first method uses the polarimetric information incompletely, and the second method uses the polarimetric information of all channels, but the operation is extensive. The final test results are shown in Fig. 11, with the green line denoting the detected wakes.
In 2002, Kasilingam et al.[91] investigated the ocean polarimetric correlation coefficient, mentioned that turbulent wakes could be observed in the image with the polarimetric correlation coefficient, and indicated that the change of surface roughness at a small scale would not modify the correlation coefficient with polarization; however, they did not state whether this parameter was beneficial to the detection of the Kelvin wake. In 2002, Morris et al.[92] carefully investigated the spatial distribution of polarimetric characteristics inside and around the sea surface of a boat’s wake and determined that, because of the nonlinear effects, the polarimetric characteristics were distributed nonuniformly in the spatial domain. When moving from the wake region to the surrounding sea area, the corresponding eigenvector distribution produces a strong transition, which helps identify the wake and clutter regions. In 2003, Morris et al.[93] proposed an entropy-based wake echo analysis method, which solved the problem of ship wake detection and analysis under low incident angle by analyzing the eigenvalue of the covariance matrix. Using the high-resolution radar system of the Defense Science and Technology Organization, the researchers obtained polarimetric measurements of wakes generated by an experiment boat operating in Port Phillip and decomposed the polarimetric covariance matrix data. The polarimetric entropy, scattering α, and anisotropy pattern figure are obtained. Then, the proper threshold is used to identify the wake and clutter regions. In 2004, Yang et al.[94] used the fully polarimetric data obtained from AIRSAR and SIR-C/X-SAR to analyze the radar features of ocean features (such as wave, oil film, ship wake, oceanfront, and seabed terrain), and based on the aforementioned analysis, determined the optimal polarization mode for observing these features. They indicated that, in most cases, HH and RL polarization is better than VV polarization. In 2006, Arnold-Bos et al.[95] proposed a multipurpose dual-base polarimetric radar simulator for sea use, including the generation of wakes. In 2011, Wu et al.[87] extracted and investigated the polarimetric characteristics of Kelvin waves based on the difference in the scattering mechanism between Kelvin wave and sea clutter in SAR images. They proposed that a simple co-polarimetric ratio can enhance the Kelvin wave, focused on the application of polarimetric decomposition, and extracted the polarimetric entropy that can more completely represent the characteristics of the Kelvin wave and provide its physical meaning. In 2018, Xu et al.[96] introduced a new two-step (rough and fine processing) detector to detect weak turbulent wakes in SAR images. Based on the polarimetric decomposition theory, this method proposed a new parameter, i.e., Surface Scattering Randomness (SSR), to enhance the difference between wake and sea surface. In the rough detection process, the potential ship wake area was extracted by the Digital Axoids Transform (DAT) of SSR. In the fine detection process, the regions obtained in the rough detection process are separated in the polarimetric domain and processed separately. Finally, regularized least squares are introduced to obtain the linear parameters of ship wakes, and prior information is obtained from the Radon transform. Experiments are conducted based on the RADARSAT-2 data, and the results show that, compared with the existing detectors based on Radon or Hough transform, the algorithm has a better capability to detect weak turbulence wakes from PolSAR images. In 2018, Biondi[97] proposed a complete process for the automatic detection of ship target motion parameters through Kelvin wakes. This method achieves clutter suppression, sparse target detection, accurate wake tendency, and Kelvin spectrum analysis by two-stage Low-Rank plus Sparse Decomposition (LRSD) combined with Radon transform. Based on the robust principal component analysis, sparse targets of interest, including ship targets and Kelvin waves, can be inferred from invariant low-rank backgrounds. In 2019, Biondi[98] considered the polarimetric information of SAR images and achieved the multichannel two-stage polarization LRSD. The upgraded algorithm achieves the extraction of polarimetric features of the sparse targets of interest and makes the detection of ship targets more accurate. The results are shown in Fig. 12. Notably, wake detection by combining sparse representation, polarization characteristics, and machine learning optimization theory will be the future trend.
In recent years, deep-learning-based algorithms have achieved great success in the field of optical target detection. The differences between the application of the deep Convolutional Neural Network (CNN) to SAR and optical images are mainly reflected by three points, i.e., the characteristic difference is significant, the data quantity is small, and the observation condition is sensitive, which are more obvious in PolSAR images. Scholars from various countries have extended these optical detection algorithms to the study of ship detection methods in SAR images, demonstrating the superior detection performance of deep learning in complex sea surface environments. The core of deep learning is to extract structure through hierarchical features, i.e., to characterize the original data with deep structures. The essence of deep learning can be regarded as a multilayer nested nonlinear fitting function, which skillfully trains the network to an appropriate fitting accuracy through a random gradient descent algorithm. The current great success of deep learning can be attributed to the emergence of the convolutional layer and the use of the Rectified Linear Unit (ReLU) activation function[100]. The ReLU is the best activation function because it is similar to neurons, i.e., neurons do not respond to some inputs, the responses of neurons to some inputs are monotonically related, and the number of neurons in the active state at any moment is always sparse. Methods represented by CNN have a flexible structure and can automatically extract structured features, which can extract not only low-dimensional features of images but also high-dimensional features of images to better identify and classify targets[100]. At present, CNN has become a research hotspot in the field of SAR ship target detection[101]. GAO et al.[102] combined binarized normed gradients and a faster region-based CNN (Faster R-CNN) to improve the detection performance. Xiaoling et al. proposed a detection method based on depthwise separable CNN for SAR ship detection with high real-time requirements, which has an obvious effect[103].
However, the publicly available literature on PolSAR ship detection using CNN is relatively limited[104–108]. In Ref. [104], the VGG network is adopted for ship detection. In Refs. [105,106], the improved Faster R-CNN is adopted. Similar to previous literature, they also adopted a network with many parameters, which easily overfits the training dataset. Cozzolino et al.[108] proposed a small network for SAR target detection. This small network can be trained directly on a limited set of SAR and PolSAR data; however, it uses the max-pooling method, which will lead to the loss of the small targets. Of course, many ship detection methods based on CNN in SAR images can be extended to PolSAR images in theory. JIN et al.[107] proposed a patch-to-pixel CNN (P2P-CNN) for small boat detection in PolSAR images, and the detection results are shown in Fig. 13. P2P-CNN shows a good performance. Fig. 13(a) illustrates the main structure of the network, which is a four-layer dense block structure with crops. The method adopts the multiplication operation combining the characteristics of each block. On the one hand, the combination yields the jump line connection effect, avoids the vanishing gradient problem, and makes the training more smooth. However, combined with multilayer semantics, the method uses high-level semantic features with powerful expression capability and the underlying semantic characteristics with only a slight change in sensitivity to jointly determine whether the center pixel is a ship and significantly reduce false alarms. Given the finiteness of PolSAR labeled data, the network parameters need to be reduced to decrease the risk of overfitting. Therefore, a dense connection module is introduced to reduce the width of the network using feature reuse, and hollow convolution is introduced to increase the sensing field and decrease the depth of the network without changing the parameters. The results show that the detection performance of P2P-CNN is considerably improved. The future trend of deep learning in PolSAR target detection is how to apply the current deep-learning-based single PolSAR image target detection methods to PolSAR images, utilize receptive field and polarimetric features, and build a new network structure.
For deep learning recognition of ship wake, large amounts of ship wake of SAR images need to be obtained for training and practice. The measured SAR images of wake are few, which do not apply to the method using deep learning. Therefore, the use of deep learning for SAR images of ship wake recognition research is relatively limited[109]. Fitch et al.[110] used the artificial neural network method to help interpret the wake after Radon transform for NASA JPL AIRSAR images. Kang used the combination of CNN and SAR images to detect the azimuth offset between the ship and the wake and investigated the potential of using the offset to automatically detect the speed of ship targets[111].
In this paper, the development and existing problems of different technical approaches for PolSAR ship detection are reviewed in detail. Table 1 lists the advantages and disadvantages of each method for the reference of interested researchers.
Detection method | Advantage | Disadvantage | ||||
Target polarimetric characteristic detection method (mainly applied to the detection of ship and clutter when the polarimetric characteri
stics are different) |
Simple polarimetric channel
synthesis technology |
The early PolSAR target dete
ction method is simple and easy to realize, and the effect is better than the single PolSAR image target detection method |
To some extent, the amplitude or phase information is used, but the polarimetric information is not fully utilized | |||
Polarimetric optimization
detection technology |
1. The information of each polari
metric channel is fully utilized 2. PolSAR images are optimized to achieve the best contrast effect |
Different optimization criteria should be selected according to the actual situation, and the selection of a sliding window also has a significant influence on the performance | ||||
Detection technology based on the
scattering mechanism |
It has strong physical interpre
tability |
Adaptability to target types and sea state needs to be improved | ||||
Spatial neighborhood detection
method |
The fusion of spatial and polarime
tric information considerably enhances the target detection capability |
Dimensions need to be reduced to avoid a dimensional disaster | ||||
Slow-moving target detection method (mainly applied to the situation where the polarimetric characteristic difference between target and clutter is small but thesre is a certain velocity diffe
rence; simultaneously, it can also extract the target motion informa tion to obtain the real-time sea surface situation) |
Single-channel PolSAR-GMTI | Cross-polarimetric information is used to enhance the detection effect of slow-moving targets | Suppression of stationary targets and detection of slow-moving targets are difficult to achieve simultaneously | |||
Virtual interferometric
slow-moving target detection |
The problem of the lack of actual interference data source is solved | It is difficult to choose the number of subaperture decompo
sition and overlap degree |
||||
Multichannel PolSAR-GMTI | Integrating the advantages of multi-platform and polarization information considerably enhan
ces the ability to move target detection |
Complex structure and cost limit engineering applications | ||||
Ship wake detection method (it is used not to detect the ship target body but to detect the wake gene
rated by the movement, mainly for small targets and stealth targets) |
Linear feature detection of
wake edge |
The detection problem is abstrac
ted into the linear feature detec tion problem, and the method is relatively simple |
It is difficult to detect different types of weak wakes in the cluttered background | |||
Background difference detection
in the wake region |
Due to the different scattering mechanisms of the wake region and background sea surface, there are considerable differences in spectrum characteristics, statisti
cal characteristics, polarimetric characteristics, and other aspects, and the effect is good |
The theoretical analysis and method implementation are more complex | ||||
Target detection algorithm based on deep learning (it has achieved great success in intelligent PolSAR image interpretation without extracting target features man
ually) |
Detection method based on CNN | Flexible structure can automa
tically extract structured features, including both low-dimensional and high-dimensional features of images |
1. It needs a large number of samples to train with
2. Interpretability needs to be investigated |
Simultaneously, with the continuous development and progress of radar technology, the future development trend of PolSAR ship target detection can be predicted as follows:
(1) At present, the optimal polarimetric technology is gradually combined with the interpretable machine learning method, and the classification optimization criterion is adopted for target detection, which may be a new method to improve the detection performance of small targets, and can be used as the development direction of subsequent polarimetric optimization detection technology. New feature vectors can be constructed from different component coefficients of different polarimetric decomposition models, and the detection performance of targets can be improved by adopting the Fisher classification criterion or other feature vector space, which is also the development trend of ship target detection based on the polarimetric decomposition theory.
(2) In the dense target environment, in addition to the pollution between the target and the clutter, some problems in the ship target group, such as splitting and merging, cause difficult image interpretation. Targeted separation of dense target obfuscation and merger of split targets are also the research direction of ship target detection in the future.
(3) The integrated system of distributed fully polarimetric radar is developed by utilizing interferometric processing technology. Interference and polarization both belong to the category of multidimensional information processing. Through the extension of spatial and polarimetric information, the detection capability of the slow and small ship targets of PolSAR can be significantly improved.
(4) There is still a contradiction between the complexity of the statistical model, the accuracy of parameter estimation, and the number of samples. The statistical model of clutter and the theory of parameter estimation are further improved. With the improvement of PolSAR resolution and the complexity of sea conditions, a uniform and universal statistical modeling framework of clutter polarimetric covariance matrix needs to be established, and its CFAR detection closed form, which will help improve the statistical theory of PolSAR ship detection, needs to be deduced.
(5) In combination with AI technologies, such as deep learning, PolSAR ship detection is driven by a small amount of data, focusing not only on the detection of ship ontology but also on the extraction of ship wake features to further enhance the ship target detection capability of PolSAR image.
[1] |
CRISP D J. The state-of-the-art in ship detection in synthetic aperture radar imagery[R]. DSTO-RR-0272, 2004.
|
[2] |
RANSON K J and SUN Guoqing. An evaluation of AIRSAR and SIR-C/X-SAR images for mapping northern forest attributes in Maine, USA[J]. Remote Sensing of Environment, 1997, 59(2): 203–222. doi: 10.1016/S0034-4257(96)00154-X
|
[3] |
代大海, 王雪松, 肖顺平, 等. PolSAR系统与技术的发展趋势[J]. 雷达科学与技术, 2008, 6(1): 15–22. doi: 10.3969/j.issn.1672-2337.2008.01.003
DAI Dahai, WANG Xuesong, and XIAO Shunping, et al. Development trend of PolSAR system and technology[J]. Radar Science and Technology, 2008, 6(1): 15–22. doi: 10.3969/j.issn.1672-2337.2008.01.003
|
[4] |
匡纲要, 陈强, 蒋咏梅, 等. 极化合成孔径雷达基础理论及其应用[M]. 长沙: 国防科技大学出版社, 2011.
KUANG Gangyao, CHEN Qiang, JIANG Yongmei, et al. Polarimetric Synthetic Aperture Radar Basic Principles and Its Applications[M]. Changsha: University of Defense Science and Technology Press, 2011.
|
[5] |
刘涛, 崔浩贵, 谢恺, 等. 极化合成孔径雷达图像解译技术[M]. 北京: 国防工业出版社, 2017.
LIU Tao, CUI Haogui, XIE Kai, et al. Interpretation Techniques in Polarimetic Synthetic Apture Radar Imagery[M]. Beijing: National Defense Industry Press, 2017.
|
[6] |
MARINO A, CLOUDE S R, and WOODHOUSE I H. Detecting depolarized targets using a new geometrical perturbation filter[J]. IEEE Transactions on Geoscience and Remote Sensing, 2012, 50(10): 3787–3799. doi: 10.1109/TGRS.2012.2185703
|
[7] |
LIU Tao, YANG Ziyuan, MARINO A, et al. PolSAR ship detection based on neighborhood polarimetric covariance matrix[J]. IEEE Transactions on Geoscience and Remote Sensing, 2020, in press. doi: 10.1109/TGRS.2020.3022181
|
[8] |
LIU Tao, YANG Ziyuan, ZHANG Tao, et al. A new form of the polarimetric notch filter[J]. IEEE Geoscience and Remote Sensing Letters, 2020, in press. doi: 10.1109/LGRS.2020.3020052
|
[9] |
SCIOTTI M, PASTINA D, and LOMBARDO P. Polarimetric detectors of extended targets for ship detection in SAR images[C]. IEEE 2001 International Geoscience and Remote Sensing Symposium Scanning the Present and Resolving the Future, Sydney, Australia, 2001.
|
[10] |
张鹏, 张嘉峰, 刘涛. 雷达多视极化检测器性能对比分析[J]. 电波科学学报, 2017, 32(4): 416–426. doi: 10.13443/j.cjors.2017022401
ZHANG Peng, ZHANG Jiafeng, and LIU Tao. Contrastive analysis of the performances of radar multi-look polarimetric detectors[J]. Chinese Journal of Radio Science, 2017, 32(4): 416–426. doi: 10.13443/j.cjors.2017022401
|
[11] |
BOERNER W M, KOSTINSKI A B, and JAMES B D. On the concept of the polarimetric matched filter in high resolution radar imaging: An alternative for speckle reduction[C]. International Geoscience and Remote Sensing Symposium, ‘Remote Sensing: Moving Toward the 21st Century’, Edinburgh, UK, 1988: 69–72.
|
[12] |
NOVAK L M, SECHTIN M B, and CARDULLO M J. Studies of target detection algorithms that use polarimetric radar data[J]. IEEE Transactions on Aerospace and Electronic Systems, 1989, 25(2): 150–165. doi: 10.1109/7.18677
|
[13] |
CHANEY R D, BUD M C, and NOVAK L M. On the performance of polarimetric target detection algorithms[J]. IEEE Aerospace and Electronic Systems Magazine, 1990, 5(11): 10–15. doi: 10.1109/62.63157
|
[14] |
NOVAK L M and BURL M C. Optimal speckle reduction in polarimetric SAR imagery[J]. IEEE Transactions on Aerospace and Electronic Systems, 1990, 26(2): 293–305. doi: 10.1109/7.53442
|
[15] |
YANG Jian, YAMAGUCHI Y, BOERNER W M, et al. Numerical methods for solving the optimal problem of contrast enhancement[J]. IEEE Transactions on Geoscience and Remote Sensing, 2000, 38(2): 965–971. doi: 10.1109/36.84197
|
[16] |
YANG Dongwen, DU Lan, LIU Hongwei, et al. Novel polarimetric contrast enhancement method based on minimal clutter to signal ratio subspace[J]. IEEE Transactions on Geoscience and Remote Sensing, 2019, 57(11): 8570–8583. doi: 10.1109/TGRS.2019.2921629
|
[17] |
NOVAK L M. Target detection studies using fully polarimetric data collected by the Lincoln Laboratory MMW SAR[C]. 92 International Conference on Radar, Brighton, UK, 1992.
|
[18] |
LIU Tao, YANG Ziyuan, YANG Jian, et al. CFAR ship detection methods using compact polarimetric SAR in a K-Wishart distribution[J]. IEEE Journal of Selected Topics in Applied Earth Observations and Remote Sensing, 2019, 12(10): 3737–3745. doi: 10.1109/JSTARS.2019.2923009
|
[19] |
LOPES A and SERY F. Optimal speckle reduction for the product model in multilook polarimetric SAR imagery and the Wishart distribution[J]. IEEE Transactions on Geoscience and Remote Sensing, 1997, 35(3): 632–647. doi: 10.1109/36.581979
|
[20] |
LIU Guoqing, HUANG Shunji, TORRE A, et al. The multilook polarimetric whitening filter (MPWF) for intensity speckle reduction in polarimetric SAR images[J]. IEEE Transactions on Geoscience and Remote Sensing, 1998, 36(3): 1016–1020. doi: 10.1109/36.673694
|
[21] |
LIU Tao, ZHANG Jiafeng, GAO Gui, et al. CFAR ship detection in polarimetric synthetic aperture radar images based on whitening filter[J]. IEEE Transactions on Geoscience and Remote Sensing, 2019, 58(1): 58–81. doi: 10.1109/TGRS.2019.2931353
|
[22] |
GAO Gui, LI Gaosheng, and LI Yipeng. Shape parameter estimator of the generalized Gaussian distribution based on the MoLC[J]. IEEE Geoscience and Remote Sensing Letters, 2018, 15(3): 350–354. doi: 10.1109/LGRS.2017.2787558
|
[23] |
GAO Gui, OUYANG Kewei, LUO Yongbo, et al. Scheme of parameter estimation for generalized gamma distribution and its application to ship detection in SAR images[J]. IEEE Transactions on Geoscience and Remote Sensing, 2017, 55(3): 1812–1832. doi: 10.1109/TGRS.2016.2634862
|
[24] |
GAO Gui, LUO Yongbo, OUYANG Kewei, et al. Statistical modeling of PMA detector for ship detection in high-resolution dual-polarization SAR images[J]. IEEE Transactions on Geoscience and Remote Sensing, 2016, 54(7): 4302–4313. doi: 10.1109/TGRS.2016.2539200
|
[25] |
GAO Gui, WANG Xiaoyang, and NIU Min. Statistical modeling of the reflection symmetry metric for sea clutter in dual-polarimetric SAR data[J]. IEEE Journal of Oceanic Engineering, 2016, 41(2): 339–345. doi: 10.1109/JOE.2015.2458231
|
[26] |
冷祥光, 计科峰, 熊博莅, 等. 面向舰船目标检测的单通道复值SAR图像统计建模方法研究[J]. 雷达学报, 2020, 9(3): 477–496. doi: 10.12000/JR20070
LENG Xiangguang, JI Kefeng, XIONG Boli, et al. Statistical modeling methods of single-channel complex-valued SAR images for ship detection[J]. Journal of Radars, 2020, 9(3): 477–496. doi: 10.12000/JR20070
|
[27] |
TAO Ding, ANFINSEN S N, and BREKKE C. Robust CFAR detector based on truncated statistics in multiple-target situations[J]. IEEE Transactions on Geoscience and Remote Sensing, 2016, 54(1): 117–134. doi: 10.1109/TGRS.2015.2451311
|
[28] |
LIU Tao, YANG Ziyuan, MARINO A, et al. Robust CFAR detector based on truncated statistics for polarimetric synthetic aperture radar[J]. IEEE Transactions on Geoscience and Remote Sensing, 2020, 58(9): 6731–6747. doi: 10.1109/TGRS.2020.2979252
|
[29] |
LANG Haitao, XI Yuyang, and ZHANG Xi. Ship detection in high-resolution SAR images by clustering spatially enhanced pixel descriptor[J]. IEEE Transactions on Geoscience and Remote Sensing, 2019, 57(8): 5407–5423. doi: 10.1109/TGRS.2019.2899337
|
[30] |
NUNZIATA F, MIGLIACCIO M, and BROWN C E. Reflection symmetry for polarimetric observation of man-made metallic targets at sea[J]. IEEE Journal of Oceanic Engineering, 2012, 37(3): 384–394. doi: 10.1109/JOE.2012.2198931
|
[31] |
WANG Na, SHI Gongtao, LIU Li, et al. Polarimetric SAR target detection using the reflection symmetry[J]. IEEE Geoscience and Remote Sensing Letters, 2012, 9(6): 1104–1108. doi: 10.1109/LGRS.2012.2189548
|
[32] |
MARINO A. A notch filter for ship detection with polarimetric SAR data[J]. IEEE Journal of Selected Topics in Applied Earth Observations and Remote Sensing, 2013, 6(3): 1219–1232. doi: 10.1109/JSTARS.2013.2247741
|
[33] |
MARINO A and HAJNSEK I. Statistical tests for a ship detector based on the polarimetric notch filter[J]. IEEE Transactions on Geoscience and Remote Sensing, 2015, 53(8): 4578–4595. doi: 10.1109/TGRS.2015.2402312
|
[34] |
GAO Gui and SHI Gongtao. CFAR ship detection in nonhomogeneous sea clutter using polarimetric SAR data based on the notch filter[J]. IEEE Transactions on Geoscience and Remote Sensing, 2017, 55(8): 4811–4824. doi: 10.1109/TGRS.2017.2701813
|
[35] |
GAO Gui and SHI Gongtao. Ship detection in dual-channel ATI-SAR based on the notch filter[J]. IEEE Transactions on Geoscience and Remote Sensing, 2017, 55(8): 4795–4810. doi: 10.1109/TGRS.2017.2701810
|
[36] |
GAO Gui, GAO Sheng, HE Juan, et al. Ship detection using compact polarimetric SAR based on the notch filter[J]. IEEE Transactions on Geoscience and Remote Sensing, 2018, 56(9): 5380–5393. doi: 10.1109/TGRS.2018.2815582
|
[37] |
CLOUDE S R and POTTIER E. A review of target decomposition theorems in radar polarimetry[J]. IEEE Transactions on Geoscience and Remote Sensing, 1996, 34(2): 498–518. doi: 10.1109/36.485127
|
[38] |
RINGROSE R and HARRIS N. Ship detection using polarimetric SAR data[J]. European Space Agency ESA SP, 2000, 450(450): 687.
|
[39] |
TOUZI R, CHARBONNEAU F, HAWKINS R K, et al. Ship-sea contrast optimization when using polarimetric SARs[C]. IEEE 2001 International Geoscience and Remote Sensing Symposium Scanning the Present and Resolving the Future, Sydney, Australia, 2001.
|
[40] |
TOUZI R. Calibrated polarimetric SAR data for ship detection[C]. IEEE 2000 International Geoscience and Remote Sensing Symposium. Taking the Pulse of the Planet: The Role of Remote Sensing in Managing the Environment. Proceedings (Cat. No. 00CH37120), Honolulu, USA, 2000.
|
[41] |
CHEN Jiong, CHEN Yilun, and YANG Jian. Ship detection using polarization cross-entropy[J]. IEEE Geoscience and Remote Sensing Letters, 2009, 6(4): 723–727. doi: 10.1109/LGRS.2009.2024224
|
[42] |
SUGIMOTO M, OUCHI K, and NAKAMURA Y. On the novel use of model-based decomposition in SAR polarimetry for target detection on the sea[J]. Remote Sensing Letters, 2013, 4(9): 843–852. doi: 10.1080/2150704X.2013.804220
|
[43] |
YANG Jian, DONG Guiwei, PENG Yingning, et al. Generalized optimization of polarimetric contrast enhancement[C]. IEEE Antennas and Propagation Society International Symposium. Digest. Held in conjunction with: USNC/CNC/URSI North American Radio Sci. Meeting (Cat. No. 03CH37450), Columbus, USA, 2003.
|
[44] |
YIN Junjun, YANG Jian, XIE Chunhua, et al. An improved generalized optimization of polarimetric contrast enhancement and its application to ship detection[J]. IEICE Transactions on Communications, 2013, E96.B(7): 2005–2013. doi: 10.1587/transcom.E96.B.2005
|
[45] |
TOUZI R, HURLEY J, and VACHON P W. Optimization of the degree of polarization for enhanced ship detection using polarimetric RADARSAT-2[J]. IEEE Transactions on Geoscience and Remote Sensing, 2015, 53(10): 5403–5424. doi: 10.1109/TGRS.2015.2422134
|
[46] |
BORDBARI R and MAGHSOUDI Y. A new target detector based on subspace projections using polarimetric SAR data[J]. IEEE Transactions on Geoscience and Remote Sensing, 2019, 57(5): 3025–3039. doi: 10.1109/TGRS.2018.2879681
|
[47] |
殷君君, 安文韬, 杨健. 基于极化散射参数与Fisher-OPCE的监督目标分类[J]. 清华大学学报: 自然科学版, 2011, 51(12): 1782–1786.
YIN Junjun, AN Wentao, and YANG Jian. Supervised target classification using polarimetric scattering parameters and Fisher-OPCE[J]. Journal of Tsinghua University:Science and Technology, 2011, 51(12): 1782–1786.
|
[48] |
CUI Xingchao, CHEN Siwei, and SU Yi. Ship detection in polarimetric SAR image based on similarity test[C]. 2019 IEEE International Geoscience and Remote Sensing Symposium, Yokohama, Japan, 2019. doi: 10.1109/IGARSS.2019.8900480.
|
[49] |
HE Jinglu, WANG Yinghua, LIU Hongwei, et al. A novel automatic PolSAR ship detection method based on superpixel-level local information measurement[J]. IEEE Geoscience and Remote Sensing Letters, 2018, 15(3): 384–388. doi: 10.1109/LGRS.2017.2789204
|
[50] |
LANG Haitao, TAO Yunhong, NIU Lihui, et al. A new scattering similarity based metric for ship detection in polarimetric synthetic aperture radar image[J]. Acta Oceanologica Sinica, 2020, 39(5): 145–150. doi: 10.1007/s13131-020-1563-7
|
[51] |
CUI Xingchao, SU Yi, and CHEN Siwei. A saliency detector for polarimetric SAR ship detection using similarity test[J]. IEEE Journal of Selected Topics in Applied Earth Observations and Remote Sensing, 2019, 12(9): 3423–3433. doi: 10.1109/JSTARS.2019.2925833
|
[52] |
HUANG Xiaojing, HUANG Pingping, DONG Lixia, et al. Saliency detection based on distance between patches in polarimetric SAR images[C]. 2014 IEEE Geoscience and Remote Sensing Symposium, Quebec City, Canada, 2014.
|
[53] |
JÄGER M and HELLWICH O. Saliency and salient region detection in SAR polarimetry[C]. 2005 IEEE International Geoscience and Remote Sensing Symposium, Seoul, South Korea, 2005.
|
[54] |
WANG Haipeng, XU Feng, and CHEN Shanshan. Saliency detector for SAR images based on pattern recurrence[J].IEEE Journal of Selected Topics in Applied Earth Observations and Remote Sensing, 2016, 9(7): 2891–2900. doi: 10.1109/JSTARS.2016.2521709
|
[55] |
LIN Huiping, CHEN Hang, JIN Kan, et al. Ship detection with superpixel-level fisher vector in high-resolution SAR images[J]. IEEE Geoscience and Remote Sensing Letters, 2020, 17(2): 247–251. doi: 10.1109/LGRS.2019.2920668
|
[56] |
ZHANG Tao, JI Jinsheng, LI Xiaofeng, et al. Ship detection from PolSAR imagery using the complete polarimetric covariance difference matrix[J]. IEEE Transactions on Geoscience and Remote Sensing, 2018, 57(5): 2824–2839. doi: 10.1109/TGRS.2018.2877821
|
[57] |
ZHANG Tao, YANG Zhen, GAN Hongping, et al. PolSAR ship detection using the joint polarimetric information[J]. IEEE Transactions on Geoscience and Remote Sensing, 2020, 58(11): 8225–8241. doi: 10.1109/TGRS.2020.2989425
|
[58] |
PERRY R P, DIPIETRO R C, and FANTE R L. SAR imaging of moving targets[J]. IEEE Transactions on Aerospace and Electronic Systems, 1999, 35(1): 188–200. doi: 10.1109/7.745691
|
[59] |
GAO Gui, WANG Xiaoyang, and LAI Tao. Detection of moving ships based on a combination of magnitude and phase in along-track interferometric SAR—Part II: Statistical modeling and CFAR detection[J]. IEEE Transactions on Geoscience and Remote Sensing, 2015, 53(7): 3582–3599. doi: 10.1109/TGRS.2014.2379351
|
[60] |
GAO Gui, WANG Xiaoyang, and LAI Tao. Detection of moving ships based on a combination of magnitude and phase in along-track interferometric SAR—Part I: SIMP metric and its performance[J]. IEEE Transactions on Geoscience and Remote Sensing, 2015, 53(7): 3565–3581. doi: 10.1109/TGRS.2014.2379352
|
[61] |
GAO Gui and SHI Gongtao. The CFAR detection of ground moving targets based on a joint metric of SAR interferogram’s magnitude and phase[J]. IEEE Transactions on Geoscience and Remote Sensing, 2012, 50(9): 3618–3624. doi: 10.1109/TGRS.2012.2184836
|
[62] |
PARK H R, LI Jian, and WANG Hong. Polarization-space-time domain generalized likelihood ratio detection of radar targets[J]. Signal Processing, 1995, 41(2): 153–164. doi: 10.1016/0165-1684(94)00097-J
|
[63] |
PARK H R, KWAG Y K, and WANG Hong. An efficient adaptive polarimetric processor with an embedded CFAR[J]. ETRI Journal, 2003, 25(3): 171–178. doi: 10.4218/etrij.03.0102.0316
|
[64] |
WANG Genyuan, XIA Xianggen, and CHEN V C. Radar imaging of moving targets in foliage using multifrequency multiaperture polarimetric SAR[J]. IEEE Transactions on Geoscience and Remote Sensing, 2003, 41(8): 1755–1764. doi: 10.1109/TGRS.2003.813501
|
[65] |
FRIEDLANDER B and PORAT B. VSAR: A high resolution radar system for detection of moving targets[J]. IEE Proceedings-Radar,Sonar and Navigation, 1997, 144(4): 205–218. doi: 10.1049/ip-rsn:19971309
|
[66] |
LIU Zhongxun, DAI Dahai, LI Dun, et al. Optimal polarimetric interferometry coherence analysis in detection and location of moving target with SAR[C]. The 1st Asian and Pacific Conference on Synthetic Aperture Radar, Huangshan, China, 2007.
|
[67] |
MATTAR K E, LIU Chen, and SABRY R. Polarimetric SAR interferometry: Investigations using EC CV-580 SAR data[R]. Ottawa: Defence Research and Development Canada Ottawa, 2005.
|
[68] |
ZOU Bin, WEI Tao, and ZHANG Lamei. Moving targets detection and analysis on multi-look polarimetric SAR images using PWF method[C]. 2008 IEEE International Geoscience and Remote Sensing Symposium, Boston, USA, 2008.
|
[69] |
LIU Chen and GIERULL C H. A new application for polsar imagery in the field of moving target indication/ship detection[J]. IEEE Transactions on Geoscience and Remote Sensing, 2007, 45(11): 3426–3436. doi: 10.1109/TGRS.2007.907192
|
[70] |
LIU Chen. Time-frequency analysis of PolSAR moving target data[J]. Canadian Journal of Remote Sensing, 2007, 33(4): 237–249. doi: 10.5589/m07-019
|
[71] |
STACY N and PREISS M. Polarimetric ATI slow target detection in a log likelihood framework[C]. 2013 IEEE International Geoscience and Remote Sensing Symposium, Melbourne, Australia, 2013.
|
[72] |
李延伟. 单/双基地极化干涉SAR信号建模、检测及参数反演方法研究[D]. [博士论文], 国防科学技术大学, 2010.
LI Yanwei. Study on the method of the modeling, the detection and the parameter inversion for the mono/bi static polarimetric SAR interferometry[D]. [Ph. D. dissertation], National University of Defense Technology, 2010.
|
[73] |
CHIU S, GIERULL C, and RASHID M. First results of experimental polarimetric SAR-GMTI modes on RADARSAT-2[C]. 2018 IEEE International Geoscience and Remote Sensing Symposium, Valencia, Spain, 2018.
|
[74] |
CHIU S, GIERULL C, and RASHID M. Ship detection, discrimination, and motion estimation via spaceborne polarimetric SAR-GMTI[C]. 2019 IEEE Radar Conference, Boston, USA, 2019.
|
[75] |
ZHANG Peng, ZHANG Jiafeng, and LIU Tao. Constant false alarm rate detection of slow targets in polarimetric along-track interferometric synthetic aperture radar imagery[J]. IET Radar,Sonar&Navigation, 2019, 13(1): 31–44. doi: 10.1049/iet-rsn.2018.5082
|
[76] |
张鹏, 张嘉峰, 刘涛. 基于相干度优化的极化顺轨干涉SAR慢小目标CFAR检测[J]. 北京航空航天大学学报, 2019, 45(3): 575–587. doi: 10.13700/j.bh.1001-5965.2018.0322
ZHANG Peng, ZHANG Jiafeng, and LIU Tao. Slow and small target CFAR detection of polarimetric along-track interferometric SAR using coherence optimization[J]. Journal of Beijing University of Aeronautics and Astronautics, 2019, 45(3): 575–587. doi: 10.13700/j.bh.1001-5965.2018.0322
|
[77] |
OUCHI K. On the multilook images of moving targets by synthetic aperture radars[J]. IEEE Transactions on Antennas and Propagation, 1985, 33(8): 823–827. doi: 10.1109/TAP.1985.1143684
|
[78] |
KIRSCHT M. Detection and velocity estimation of moving objects in a sequence of single-look SAR images[C]. 1996 International Geoscience and Remote Sensing Symposium, Lincoln, USA, 1996.
|
[79] |
GAO Fei, MAO Shiyi, SUN Jinping, et al. A tri-look detector for single channel SAR-GMTI[C]. 2009 IET International Radar Conference, Guilin, China, 2009.
|
[80] |
康雪艳, 杨汝良. 利用DPCA方法对机载单天线SAR实际数据进行动目标检测[J]. 遥感技术与应用, 2004, 19(3): 182–186. doi: 10.3969/j.issn.1004-0323.2004.03.009
KANG Xueyan and YANG Ruliang. Moving targets detection using DPCA Method for single-antenna airborne SAR real data[J]. Remote Sensing Technology and Application, 2004, 19(3): 182–186. doi: 10.3969/j.issn.1004-0323.2004.03.009
|
[81] |
张露, 郭华东, 韩春明, 等. 基于子孔径分解的SAR动目标检测方法[J]. 电子学报, 2008, 36(6): 1210–1213. doi: 10.3321/j.issn:0372-2112.2008.06.034
ZHANG Lu, GUO Huadong, HAN Chunming, et al. Moving targets detection in SAR images based on sub-aperture decomposition[J]. Acta Electronica Sinica, 2008, 36(6): 1210–1213. doi: 10.3321/j.issn:0372-2112.2008.06.034
|
[82] |
康雪艳, 杨汝良. 对机载单天线SAR实际数据进行ATI动目标检测的新方法[J]. 电子学报, 2005, 33(3): 416–418. doi: 10.3321/j.issn:0372-2112.2005.03.008
KANG Xueyan and YANG Ruliang. A new method of moving target detection using ATI for single-antenna airborne SAR real data[J]. Acta Electronica Sinica, 2005, 33(3): 416–418. doi: 10.3321/j.issn:0372-2112.2005.03.008
|
[83] |
MARINO A, SANJUAN-FERRER M J, HAJNSEK I, et al. Ship detection with spectral analysis of synthetic aperture radar: A comparison of new and well-known algorithms[J]. Remote Sensing, 2015, 7(5): 5416–5439. doi: 10.3390/rs70505416
|
[84] |
种劲松, 朱敏慧. SAR图像舰船及其尾迹检测研究综述[J]. 电子学报, 2003, 31(9): 1356–1360. doi: 10.3321/j.issn:0372-2112.2003.09.020
CHONG Jinsong and ZHU Minhui. Survey of the study on ship and wake detection in SAR imagery[J]. Acta Electronica Sinica, 2003, 31(9): 1356–1360. doi: 10.3321/j.issn:0372-2112.2003.09.020
|
[85] |
SCHULER D L, LEE J S, HOPPEL K, et al. Polarimetric sar image signatures of gulf-stream features and ship wakes[C]. 1992 International Geoscience and Remote Sensing Symposium, Houston, USA, 1992.
|
[86] |
POTTIER E, BOERNER W M, and SCHULER D L. Polarimetric detection and estimation of ship wakes[C]. IEEE 1999 International Geoscience and Remote Sensing Symposium, Hamburg, Germany, 1999.
|
[87] |
WU Peng, WANG Jun, WANG Wenguang, et al. Polarimetric characters extraction research of Kelvin wakes on PolSAR image[C]. 2011 International Conference on Remote Sensing, Environment and Transportation Engineering, Nanjing, China, 2011.
|
[88] |
HENNINGS I, ROMEISER R, ALPERS W, et al. Radar imaging of Kelvin arms of ship wakes[J]. International Journal of Remote Sensing, 1999, 20(13): 2519–2543. doi: 10.1080/014311699211912
|
[89] |
叶文隽. SAR图像舰船尾迹检测研究[D]. [硕士论文], 国防科学技术大学, 2009.
YE Wenjun. Research on detection of ship wake from SAR imagery[D]. [Master dissertation], National University of Defense Technology, 2009.
|
[90] |
IMBO P, SOUYRIS J C, and YEREMY M. Wake detection in polarimetric SAR images[C]. IEEE 2001 International Geoscience and Remote Sensing Symposium Scanning the Present and Resolving the Future, Sydney, Australia, 2001.
|
[91] |
KASILINGAM D, SCHULER D, LEE J S, et al. Modulation of polarimetric coherence by ocean features[C]. 2002 IEEE International Geoscience and Remote Sensing Symposium, Toronto, Canada, 2002: 432–434.
|
[92] |
MORRIS J, ANDERSON S, and PARFITT A. Polarimetric mapping of ship wakes[C]. 2002 IEEE International Geoscience and Remote Sensing Symposium, Toronto, Canada, 2002.
|
[93] |
MORRIS J and ANDERSON S. An entropy-based approach to wake echo analysis [ship wake radar detection][C]. 2003 International Conference on Radar, Adelaide, Australia, 2003.
|
[94] |
YANG Jingsong, HUANG Weigen, XIAO Qingmei, et al. Optimal polarization for the observation of ocean features with SAR[C]. 2004 IEEE International Geoscience and Remote Sensing Symposium, Anchorage, USA, 2004.
|
[95] |
ARNOLD-BOS A, MARTIN A, and KHENCHAF A. A versatile bistatic & polarimetric marine radar simulator[C]. 2006 IEEE Conference on Radar, Verona, USA, 2006.
|
[96] |
XU Zhou, TANG Bo, and CHENG Shuiying. Faint ship wake detection in PolSAR images[J]. IEEE Geoscience and Remote Sensing Letters, 2018, 15(7): 1055–1059. doi: 10.1109/LGRS.2018.2823007
|
[97] |
BIONDI F. Low-rank plus sparse decomposition and localized radon transform for ship-wake detection in synthetic aperture radar images[J]. IEEE Geoscience and Remote Sensing Letters, 2018, 15(1): 117–121. doi: 10.1109/LGRS.2017.2777264
|
[98] |
BIONDI F. A polarimetric extension of low-rank plus sparse decomposition and radon transform for ship wake detection in synthetic aperture radar images[J]. IEEE Geoscience and Remote Sensing Letters, 2019, 16(1): 75–79. doi: 10.1109/LGRS.2018.2868365
|
[99] |
徐丰, 王海鹏, 金亚秋, 等. 合成孔径雷达图像智能解译[M]. 北京: 科学出版社, 2020.
XU Feng, WANG Haipeng, JIN Yaqiu, et al. Synthetic Aperture Radar Image Intelligent Interpretation[M]. Beijing: Science Press, 2020.
|
[100] |
SAIN S R. The nature of statistical learning theory[J]. Technometrics, 1996, 38(4): 409. doi: 10.1080/00401706.1996.10484565
|
[101] |
杜兰, 王兆成, 王燕, 等. 复杂场景下单通道SAR目标检测及鉴别研究进展综述[J]. 雷达学报, 2020, 9(1): 34–54. doi: 10.12000/JR19104
DU Lan, WANG Zhaocheng, WANG Yan, et al. Survey of research progress on target detection and discrimination of single-channel SAR images for complex scenes[J]. Journal of Radars, 2020, 9(1): 34–54. doi: 10.12000/JR19104
|
[102] |
GAO Gui. A parzen-window-kernel-based CFAR algorithm for ship detection in SAR images[J]. IEEE Geoscience and Remote Sensing Letters, 2011, 8(3): 557–561. doi: 10.1109/LGRS.2010.2090492
|
[103] |
张晓玲, 张天文, 师君, 等. 基于深度分离卷积神经网络的高速高精度SAR舰船检测[J]. 雷达学报, 2019, 8(6): 841–851. doi: 10.12000/JR19111
ZHANG Xiaoling, ZHANG Tianwen, SHI Jun, et al. High-speed and high-accurate SAR ship detection based on a depthwise separable convolution neural network[J]. Journal of Radars, 2019, 8(6): 841–851. doi: 10.12000/JR19111
|
[104] |
CHEN Siwei, TAO Chensong, WANG Xuesong, et al. Polarimetric SAR targets detection and classification with deep convolutional neural network[C]. 2018 Progress in Electromagnetics Research Symposium, Toyama, Japan, 2018.
|
[105] |
ZHOU Feng, FAN Weiwei, SHENG Qiangqiang, et al. Ship detection based on deep convolutional neural networks for polsar images[C]. 2018 IEEE International Geoscience and Remote Sensing Symposium, Valencia, Spain, 2018.
|
[106] |
鲁兵兵. 基于深度学习的PolSAR图像分类与舰船检测方法[D]. [硕士论文], 西安电子科技大学, 2019.
LU Bingbing. PolSAR image classification and ship detection method based on deep learning[D]. [Master dissertation], Xidian University, 2019.
|
[107] |
JIN Kan, CHEN Yilun, XU Bin, et al. A patch-to-pixel convolutional neural network for small ship detection with PolSAR images[J]. IEEE Transactions on Geoscience and Remote Sensing, 2020, 58(9): 6623–6638. doi: 10.1109/TGRS.2020.2978268
|
[108] |
COZZOLINO D, DI MARTINO G, POGGI G, et al. A fully convolutional neural network for low-complexity single-stage ship detection in sentinel-1 SAR images[C]. 2017 IEEE International Geoscience and Remote Sensing Symposium, Fort Worth, USA, 2017: 886889.
|
[109] |
李其. 基于深度特征的SAR图像舰船目标检测方法研究[D]. [硕士论文], 电子科技大学, 2020.
LI Qi. Research of ship detection in SAR images based on depth features[D]. [Master dissertation], University of Electronic Science and Technology of China, 2020.
|
[110] |
FITCH J P, LEHMAN S K, DOWLA F U, et al. Ship wake-detection procedure using conjugate gradient trained artificial neural networks[J]. IEEE Transactions on Geoscience and Remote Sensing, 1991, 29(5): 718–726. doi: 10.1109/36.83986
|
[111] |
KANG K M and KIM D J. Ship velocity estimation from ship wakes detected using convolutional neural networks[J]. IEEE Journal of Selected Topics in Applied Earth Observations and Remote Sensing, 2019, 12(11): 4379–4388. doi: 10.1109/JSTARS.2019.2949006
|
[1] | YIN Junjun, LUO Jiahao, LI Xiang, DAI Xiaokang, YANG Jian. Ship Detection Based on Polarimetric SAR Gradient and Complex Wishart Classifier[J]. Journal of Radars, 2024, 13(2): 396-410. doi: 10.12000/JR23198 |
[2] | CUI Xingchao, SU Yi, CHEN Siwei. Polarimetric SAR Ship Detection Based on Polarimetric Rotation Domain Features and Superpixel Technique[J]. Journal of Radars, 2021, 10(1): 35-48. doi: 10.12000/JR20147 |
[3] | ZHU Qingtao, YIN Junjun, ZENG Liang, YANG Jian. Polarimetric SAR Image Affine Registration Based on Neighborhood Consensus[J]. Journal of Radars, 2021, 10(1): 49-60. doi: 10.12000/JR20120 |
[4] | PANG Lei, ZHANG Fengli, WANG Guojun, LIU Na, SHAO Yun, ZHANG Jiameng, ZHAO Yuchuan, PANG Lei. Imaging Simulation and Damage Assessment Feature Analysis of Ku Band Polarized SAR of Buildings[J]. Journal of Radars, 2020, 9(3): 578-587. doi: 10.12000/JR20061 |
[5] | QIN Xianxiang, YU Wangsheng, WANG Peng, CHEN Tianping, ZOU Huanxin. Weakly Supervised Classification of PolSAR Images Based on Sample Refinement with Complex-Valued Convolutional Neural Network[J]. Journal of Radars, 2020, 9(3): 525-538. doi: 10.12000/JR20062 |
[6] | DENG Yunkai, YU Weidong, ZHANG Heng, WANG Wei, LIU Dacheng, WANG Robert. Forthcoming Spaceborne SAR Development[J]. Journal of Radars, 2020, 9(1): 1-33. doi: 10.12000/JR20008 |
[7] | LI Yongzhen, HUANG Datong, XING Shiqi, WANG Xuesong. A Review of Synthetic Aperture Radar Jamming Technique[J]. Journal of Radars, 2020, 9(5): 753-764. doi: 10.12000/JR20087 |
[8] | LENG Xiangguang, JI Kefeng, XIONG Boli, KUANG Gangyao. Statistical Modeling Methods of Single-channel Complex-valued SAR Images for Ship Detection[J]. Journal of Radars, 2020, 9(3): 477-496. doi: 10.12000/JR20070 |
[9] | CHEN Huiyuan, LIU Zeyu, GUO Weiwei, ZHANG Zenghui, YU Wenxian. Fast Detection of Ship Targets for Large-scale Remote Sensing Image Based on a Cascade Convolutional Neural Network[J]. Journal of Radars, 2019, 8(3): 413-424. doi: 10.12000/JR19041 |
[10] | ZHANG Lamei, ZHANG Siyu, DONG Hongwei, ZHU Sha. Robust Classification of PolSAR Images Based on Pinball loss Support Vector Machine[J]. Journal of Radars, 2019, 8(4): 448-457. doi: 10.12000/JR19055 |
[11] | ZHANG Xiangrong, YU Xinyuan, TANG Xu, HOU Biao, JIAO Licheng. PolSAR Image Classification Method Based on Markov Discriminant Spectral Clustering[J]. Journal of Radars, 2019, 8(4): 425-435. doi: 10.12000/JR19059 |
[12] | Chen Siwei, Li Yongzhen, Wang Xuesong, Xiao Shunping. Polarimetric SAR Target Scattering Interpretation in Rotation Domain: Theory and Application[J]. Journal of Radars, 2017, 6(5): 442-455. doi: 10.12000/JR17033 |
[13] | Zou Huanxin, Luo Tiancheng, Zhang Yue, Zhou Shilin. Combined Conditional Random Fields Model for Supervised PolSAR Images Classification[J]. Journal of Radars, 2017, 6(5): 541-553. doi: 10.12000/JR16109 |
[14] | Yang Wen, Zhong Neng, Yan Tianheng, Yang Xiangli. Classification of Polarimetric SAR Images Based on the Riemannian Manifold[J]. Journal of Radars, 2017, 6(5): 433-441. doi: 10.12000/JR17031 |
[15] | Liu Zeyu, Liu Bin, Guo Weiwei, Zhang Zenghui, Zhang Bo, Zhou Yueheng, Ma Gao, Yu Wenxian. Ship Detection in GF-3 NSC Mode SAR Images[J]. Journal of Radars, 2017, 6(5): 473-482. doi: 10.12000/JR17059 |
[16] | Zhang Jie, Zhang Xi, Fan Chenqing, Meng Junmin. Discussion on Application of Polarimetric Synthetic Aperture Radar in Marine Surveillance[J]. Journal of Radars, 2016, 5(6): 596-606. doi: 10.12000/JR16124 |
[17] | Hong Wen. Hybrid-polarity Architecture Based Polarimetric SAR: Principles and Applications (in English)[J]. Journal of Radars, 2016, 5(6): 559-595. doi: 10.12000/JR16074 |
[18] | Xing Yanxiao, Zhang Yi, Li Ning, Wang Yu, Hu Guixiang. Polarimetric SAR Image Supervised Classification Method Integrating Eigenvalues[J]. Journal of Radars, 2016, 5(2): 217-227. doi: 10.12000/JR16019 |
[19] | Ji Kefeng, Wang Haibo, Leng Xiangguang, Xing Xiangwei, Kang Lihong. Spaceborne Compact Polarimetric Synthetic Aperture Radar for Ship Detection[J]. Journal of Radars, 2016, 5(6): 607-619. doi: 10.12000/JR16083 |
[20] | Hua Wen-qiang, Wang Shuang, Hou Biao. Semi-supervised Learning for Classification of Polarimetric SAR Images Based on SVM-Wishart[J]. Journal of Radars, 2015, 4(1): 93-98. doi: 10.12000/JR14138 |
1. | 师俞晨. 基于遥感影像水下目标尾迹探测综述. 现代防御技术. 2024(01): 83-91 . ![]() | |
2. | 李煜,杨静飞,张鸿生,李刚,陈杰. 极化合成孔径雷达遥感地物分类研究进展. 遥感学报. 2024(08): 1835-1853 . ![]() | |
3. | 周慧,朱虹,陈澎. 基于可变形的多尺度自注意力特征融合SAR影像舰船识别. 大连海事大学学报. 2024(04): 110-118 . ![]() | |
4. | 林晓晶,肖鹏浩,何良,王海鹏. 基于极化神经网络的雷达舰船检测识别方法. 上海航天(中英文). 2023(01): 53-60 . ![]() | |
5. | 邢世其,全斯农,范晖,王威,黄大通,李永祯,王雪松. 联合数学规划策略和精细极化分解的极化SAR舰船目标检测. 中国科学:信息科学. 2023(03): 585-605 . ![]() | |
6. | 向诚,颜世杰,桂玲. 不平衡SAR图像舰船目标识别模型. 舰船科学技术. 2023(05): 174-177 . ![]() | |
7. | 曹运运,杨子渊,刘维建,刘涛. 雷达极化对角加载检测器的最优权重算法. 雷达科学与技术. 2023(02): 222-230 . ![]() | |
8. | 罗嘉豪,殷君君,杨健. 基于超像素与稀疏重构显著性的极化SAR舰船检测. 工程科学学报. 2023(10): 1684-1692 . ![]() | |
9. | 李郝亮,陈思伟. 海面角反射体电磁散射特性与雷达鉴别研究进展与展望. 雷达学报. 2023(04): 738-761 . ![]() | |
10. | 王春华,王方超. 基于改进阈值函数的SAR图像小波去噪方法. 微电子学与计算机. 2022(05): 39-44 . ![]() | |
11. | 王少博,张成,苏迪,冀瑞静. 基于改进YOLOv3和核相关滤波算法的旋转弹目标探测算法. 兵工学报. 2022(05): 1032-1045 . ![]() | |
12. | 王志鹤,行坤,崔宁,喻忠军. 一种基于Radon变换和尾迹模型的尾迹检测算法. 电子设计工程. 2022(12): 1-6 . ![]() | |
13. | 任吉宏,刘畅. 基于自适应超像素的少样本极化SAR图像特征增强方法研究. 电子技术应用. 2022(10): 144-149 . ![]() | |
14. | 张阳,刘小芳,周鹏成. 改进Faster R-CNN的SAR图像船舶检测技术. 无线电工程. 2022(12): 2280-2287 . ![]() | |
15. | 常佳慧,赵建辉,李宁. 一种改进的2P-CFAR SAR舰船检测方法. 国外电子测量技术. 2021(11): 7-12 . ![]() |
Detection method | Advantage | Disadvantage | ||||
Target polarimetric characteristic detection method (mainly applied to the detection of ship and clutter when the polarimetric characteri
stics are different) |
Simple polarimetric channel
synthesis technology |
The early PolSAR target dete
ction method is simple and easy to realize, and the effect is better than the single PolSAR image target detection method |
To some extent, the amplitude or phase information is used, but the polarimetric information is not fully utilized | |||
Polarimetric optimization
detection technology |
1. The information of each polari
metric channel is fully utilized 2. PolSAR images are optimized to achieve the best contrast effect |
Different optimization criteria should be selected according to the actual situation, and the selection of a sliding window also has a significant influence on the performance | ||||
Detection technology based on the
scattering mechanism |
It has strong physical interpre
tability |
Adaptability to target types and sea state needs to be improved | ||||
Spatial neighborhood detection
method |
The fusion of spatial and polarime
tric information considerably enhances the target detection capability |
Dimensions need to be reduced to avoid a dimensional disaster | ||||
Slow-moving target detection method (mainly applied to the situation where the polarimetric characteristic difference between target and clutter is small but thesre is a certain velocity diffe
rence; simultaneously, it can also extract the target motion informa tion to obtain the real-time sea surface situation) |
Single-channel PolSAR-GMTI | Cross-polarimetric information is used to enhance the detection effect of slow-moving targets | Suppression of stationary targets and detection of slow-moving targets are difficult to achieve simultaneously | |||
Virtual interferometric
slow-moving target detection |
The problem of the lack of actual interference data source is solved | It is difficult to choose the number of subaperture decompo
sition and overlap degree |
||||
Multichannel PolSAR-GMTI | Integrating the advantages of multi-platform and polarization information considerably enhan
ces the ability to move target detection |
Complex structure and cost limit engineering applications | ||||
Ship wake detection method (it is used not to detect the ship target body but to detect the wake gene
rated by the movement, mainly for small targets and stealth targets) |
Linear feature detection of
wake edge |
The detection problem is abstrac
ted into the linear feature detec tion problem, and the method is relatively simple |
It is difficult to detect different types of weak wakes in the cluttered background | |||
Background difference detection
in the wake region |
Due to the different scattering mechanisms of the wake region and background sea surface, there are considerable differences in spectrum characteristics, statisti
cal characteristics, polarimetric characteristics, and other aspects, and the effect is good |
The theoretical analysis and method implementation are more complex | ||||
Target detection algorithm based on deep learning (it has achieved great success in intelligent PolSAR image interpretation without extracting target features man
ually) |
Detection method based on CNN | Flexible structure can automa
tically extract structured features, including both low-dimensional and high-dimensional features of images |
1. It needs a large number of samples to train with
2. Interpretability needs to be investigated |