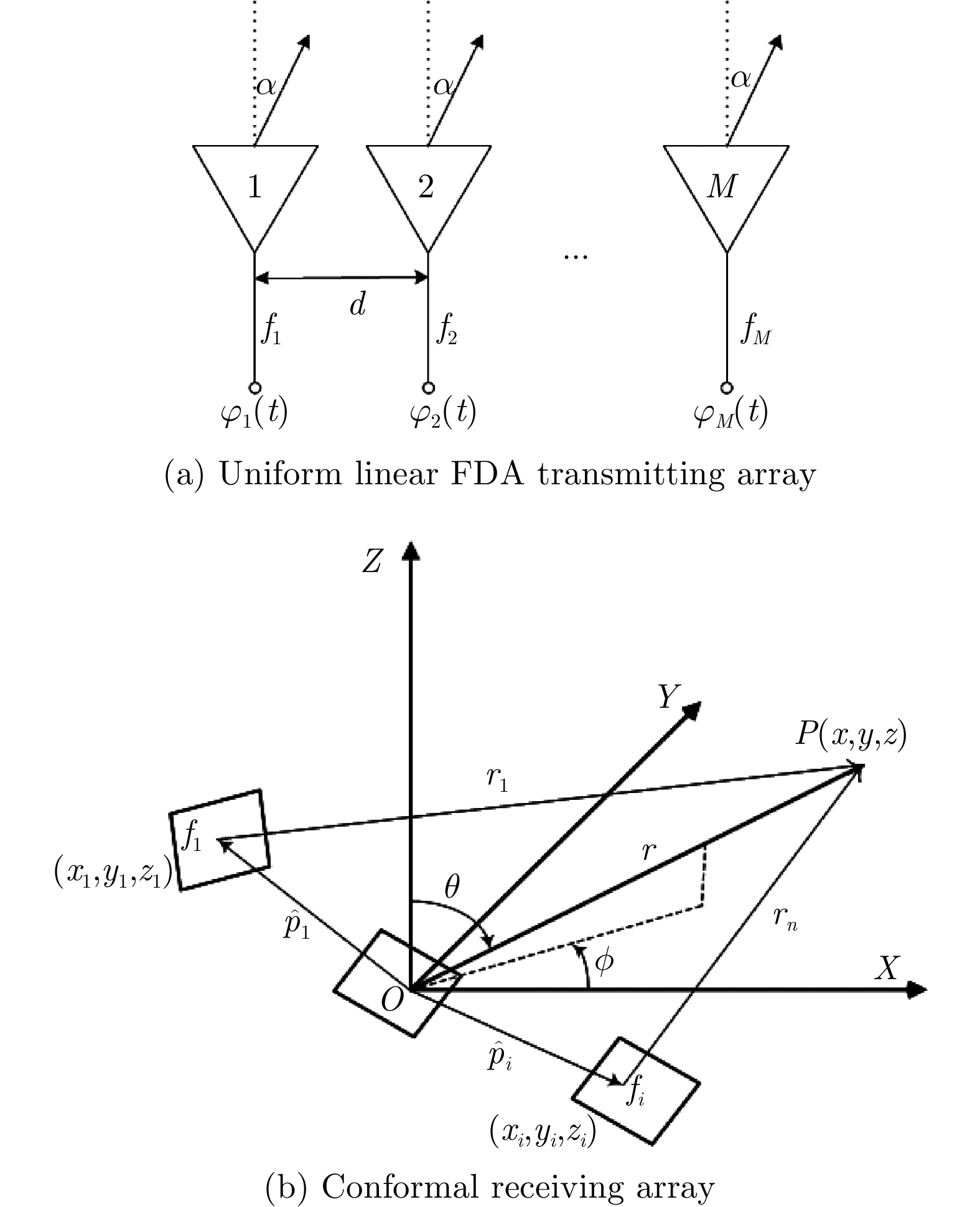
Citation: | MOU Xiaoqian, CHEN Xiaolong, GUAN Jian, et al. Clutter suppression and marine target detection for radar images based on INet[J]. Journal of Radars, 2020, 9(4): 640–653. doi: 10.12000/JR20090 |
In recent years, Frequency Diverse Array (FDA) radar has received much attention due to its range-angle-time-dependent beampattern[1,2]. Combining the advantages of FDA and traditional phased array Multiple-Input Multiple-Output (MIMO) radar in the degree of freedom, the FDA Multiple-Input Multiple Output (FDA-MIMO) radar was proposed in Ref. [3] and applied in many fields[4-9]. For parameter estimation algorithm, the authors first proposed a FDA-MIMO target localization algorithm based on sparse reconstruction theory[10], and an unbiased joint range and angle estimation method was proposed in Ref. [11]. The work of Ref. [12] further proved that the FDA-MIMO is superior to traditional MIMO radar in range and angle estimation performance, and the authors of Ref. [13] introduced a super-resolution MUSIC algorithm for target location, and analyzed its resolution threshold. Meanwhile, high-resolution Doppler processing is utilized for moving target parameter estimation[14]. The Estimation of Signal Parameters via Rotational Invariance Technique (ESPRIT) and PARAllel FACtor (PARAFAC) was proposed in Ref. [15], which is a search-free algorithm for FDA-MIMO.
Moreover, the research of conformal array has received more and more attention. Conformal array is a non-planar array that can be completely attached to the surface of the carrier[16]. It has significant advantages such as reducing the aerodynamic impact on the carrier and smaller radar cross section[17]. In addition, conformal array can achieve wide-angle scanning with a lower SideLobe Level (SLL)[18]. Different from traditional arrays, the element beampattern of conformal array needs to be modeled separately in the parameter estimation due to the difference of carrier curvature[19-21].
As far as we know, most of the existing researches on FDA-MIMO are based on linear array, while there is little research on the combination of FDA-MIMO and conformal array[22]. In this paper, we replace the receiving array in the traditional FDA-MIMO with conformal array. Compared with conventional FDA-MIMO, conformal FDA-MIMO inherits the merits of conformal array and FDA-MIMO, which can effectively improve the stealth and anti-stealth performance of the carrier, and reduce the volume and the air resistance of the carrier. For conformal FDA-MIMO, we further study the parameters estimation algorithm. The major contributions of this paper are summarized as follows:
(1) A conformal FDA-MIMO radar model is first formulated.
(2) The parameter estimation Cramér-Rao Lower Bound (CRLB) for conformal FDA-MIMO radar is derived.
(3) Inspired by the existing work of Refs. [23,24], a Reduced-Dimension MUSIC (RD-MUSIC) algorithm for conformal FDA-MIMO radar is correspondingly proposed to reduce the complexity.
The rest of the paper consists of four parts. Section 2 formulates the conformal FDA-MIMO radar model, and Section 3 derives a RD-MUSIC algorithm for conformal FDA-MIMO radar. Simulation results for conformal FDA-MIMO radar with semi conical conformal receiving array are provided in Section 4. Finally, conclusions are drawn in Section 5.
For the convenience of analysis, we consider a monostatic conformal FDA-MIMO radar which is composed by a
The complex envelope of the transmitted signal of the mth transmitting element is denoted as
∫Tpφm(t)φ∗m1(t−τ)dt=0,m1≠m | (1) |
where
sm(t)=am(t,θ,ϕ,r)φm(t),0≤t≤Tp | (2) |
where
am(t,θ,ϕ,r)=exp{−j2π((m−1)Δfrc−f1(m−1)dsinαc−(m−1)Δft)} | (3) |
is the mth element of the transmitting steering vector according to the phase difference between adjacent elements, the angle between far-field target and transmitting array is denoted as
Δψt0=2π(Δfrc−f1dsinαc−Δft) | (4) |
where
a0(t,θ,ϕ,r)=[1,exp{−jΔψt0},⋯,exp{−j(M−1)Δψt0}]T | (5) |
For the conformal receiving array, as shown in Fig. 1(b), the time delay between target
τn=rn/c | (6) |
where
rn≈r−→pn⋅→r | (7) |
where r denotes the range between the target and the origin point,
Δτrn=τ1−τn=u(xn−x1)+v(yn−y1)+cosθ(zn−z1)c | (8) |
And the corresponding phase difference between the first element and the nth element is
ΔψRn=2πf1Δτrn | (9) |
Consequently, the receiving steering vector is
b(θ,ϕ)=[r1(θ,ϕ),r2(θ,ϕ)exp(jΔψr2),⋯,rN(θ,ϕ)exp(jΔψrN)]T | (10) |
where
Then the total phase difference between adjacent transmitting array elements can be rewritten as
Δψt=2π(Δf2rc−f1dsinαc−Δft) | (11) |
where the factor
a(t,θ,ϕ,r)=[1,exp{−jΔψt},⋯,exp{−j(M−1)Δψt}]T | (12) |
Assuming L far-field targets are located at
X=AS+N | (13) |
where the array manifold
A=[at,r(θ1,ϕ1,r1),⋯,at,r(θL,ϕL,rL)]=[b(θ1,ϕ1)⊗a(θ1,ϕ1,r1),⋯,b(θL,ϕL)⊗a(θL,ϕL,rL)] | (14) |
where
a(θ,ϕ,r)=[1exp{−j2π(2Δfrc−f1dsinαc)}⋯exp{−j2π(M−1)(2Δfrc−f1dsinαc)}] | (15) |
which can be expressed as
a(θ,ϕ,r)=a(θ,ϕ)⊙a(r) | (16) |
where
a(r)=[1,exp(−j2π2Δfrc),⋯,exp(−j2π(M−1)2Δfrc)]T | (17) |
a(θ,ϕ)=[1,exp(j2πf1dsinαc),⋯,exp[j2π(M−1)f1dsinαc]]T | (18) |
and
The CRLB can be obtained from the inverse of Fisher information matrix[27,28], which establishes a lower bound for the variance of any unbiased estimator. We employ the CRLB for conformal FDA-MIMO parameter estimation to evaluate the performance of some parameter estimation algorithms.
The discrete signal model is
x[k]=at,r(θ,ϕ,r)s[k]+N[k],k=1,2,⋯,K | (19) |
For the sake of simplification, we take
The Probability Distribution Function (PDF) of the signal model with
p(x|θ,ϕ,r)=1(2πσ2n)K2⋅exp(−1σ2n(x−at,rs)H(x−at,rs)) | (20) |
where
The CRLB matrix form of elevation angle, azimuth angle and range is given by Eq. (21), diagonal elements
CRLB=[CθθCθϕCθrCϕθCϕϕCϕrCrθCrϕCrr]=FIM−1=[F11F12F13F21F22F23F31F32F33] | (21) |
The elements of Fisher matrix can be expressed as
Fij=−E[∂2ln(p(x∣θ,ϕ,r))∂xi∂xj],i,j=1,2,3 | (22) |
In the case of
p(x|θ,ϕ,r)=Cexp{−1σ2nK∑n=1(x[k]−at,rs[k])H⋅(x[k]−at,rs[k])} | (23) |
where
ln(p(x|θ,ϕ,r))=ln(C)−1σ2nK∑k=1(x[k]−at,rs[k])H⋅(x[k]−at,rs[k]) | (24) |
where
F11=−E[∂2ln(p(x|θ,ϕ,r))∂θ2] | (25) |
Correspondingly, the first derivative of natural logarithm is given by
∂ln(p(x|θ,ϕ,r))∂θ=−1σ2nK∑k=1(−xH[k]∂at,r∂θs[k]−∂aHt,r∂θs[k]x[k]+∂aHt,r∂θat,rs2[n]a+aHt,r∂at,r∂θs2[n]) | (26) |
Then we can obtain the second derivative of
∂2ln(p(x|θ,ϕ,r))∂θ2=−1σ2nK∑k=1(−x[k]H∂2at,r∂θ2s[k]−∂2aHt,r∂θ2s(k)x[k]+∂2aHt,r∂θ2at,rs[k]2+∂aHt,r∂θ∂at,r∂θs[k]2+∂aHt,r∂θ∂at,r∂θs[k]2+aHt,r∂2at,r∂θ2s[k]2) | (27) |
And then we have
K∑k=1x[k]=K∑k=1at,rs[k]+N[k]=at,r(θ,ϕ,r)K∑k=1s[k] | (28) |
and
K∑k=1s2[k]=Kvar(s[k])=Kσ2s | (29) |
where
E[∂2ln(p(x|θ,ϕ,r))∂θ2]=−Kσ2sσ2n(∂aHt,r∂θ∂at,r∂θ+∂aHt,r∂θ∂at,r∂θ)=−2Kσ2sσ2n‖∂at,r∂θ‖2 | (30) |
where
CRLB−1=FIM=2Kσ2sσ2n⋅[‖∂a∂θ‖2FIM12FIM13FIM21‖∂a∂ϕ‖2FIM23FIM31FIM32‖∂a∂r‖2] | (31) |
where
FIM12=12[∂aHt,r∂θ∂at,r∂ϕ+∂aHt,r∂ϕ∂at,r∂θ], |
FIM13=12[∂aHt,r∂θ∂at,r∂r+∂aHt,r∂r∂at,r∂θ], |
FIM21=12[∂aHt,r∂ϕ∂at,r∂θ+∂aHt,r∂θ∂at,r∂ϕ], |
FIM23=12[∂aHt,r∂ϕ∂at,r∂r+∂aHt,r∂r∂at,r∂ϕ], |
FIM31=12[∂aHt,r∂r∂at,r∂θ+∂aHt,r∂θ∂at,r∂r], |
FIM32=12[∂aHt,r∂r∂at,r∂ϕ+∂aHt,r∂ϕ∂at,r∂r], |
σ2sσ2n=SNR |
Finally, the CRLB of conformal FDA-MIMO can be calculated by the inverse of Fisher matrix.
The covariance matrix of the conformal FDA-MIMO receiving signal can be written as
RX=ARsAH+σ2IMN | (32) |
where
RX=USΛSUHS+UnΛnUHn | (33) |
The traditional MUSIC algorithm is utilized to estimate the three-dimensional parameters
PMUSIC(θ,ϕ,r)=1aHt,r(θ,ϕ,r)UnUHnat,r(θ,ϕ,r) | (34) |
The target location can be obtained by mapping the peak indexes of MUSIC spectrum.
Traditional MUSIC parameter estimation algorithm is realized by 3D parameter search, which has good performance at the cost of high computational complexity. When the angular scan interval is less than 0.1°, the running time of single Monte-Carlo simulation is in hours, which is unpracticable for us to analysis conformal FDA-MIMO estimation performance by hundreds of simulations.
In order to reduce the computation complexity of the parameter estimation algorithm for conformal FDA-MIMO, we propose a RD-MUSIC algorithm, which has a significant increase in computing speed at the cost of little estimation performance loss.
At first, we define
V(θ,ϕ,r)=aHt,r(θ,ϕ,r)HUnUHnat,r(θ,ϕ,r)=[b(θ,ϕ)⊗a(θ,ϕ,r)]HUn⋅UHn[b(θ,ϕ)⊗a(θ,ϕ,r)] | (35) |
Eq. (35) can be further calculated by
V(θ,ϕ,r)=aH(θ,ϕ,r)[b(θ,ϕ)⊗IM]H×UnUHn[b(θ,ϕ)⊗IM]a(θ,ϕ,r)=aH(θ,ϕ,r)Q(θ,ϕ)a(θ,ϕ,r) | (36) |
where
Eq. (36) can be transformed into a quadratic programming problem. To avoid
{minθ,ϕ,r aH(θ,ϕ,r)Q(θ,ϕ)a(θ,ϕ,r)s.t. eH1a(θ,ϕ,r)=1 | (37) |
The penalty function can be constructed as
L(θ,ϕ,r)=aH(θ,ϕ,r)Q(θ,ϕ)a(θ,ϕ,r)−μ(eH1a(θ,ϕ,r)−1) | (38) |
where
∂L(θ,ϕ,r)∂a(r)=2diag{a(θ,ϕ)}Q(θ,ϕ)a(θ,ϕ,r)−μdiag{a(θ,ϕ)}e1 | (39) |
where
And then let
a(r)=ςQ−1(θ,ϕ)e1./a(θ,ϕ) | (40) |
where
a(r)=Q−1(θ,ϕ)e1eH1Q−1(θ,ϕ)e1./a(θ,ϕ) | (41) |
Substituting
<ˆθ,ˆϕ>=argminθ,ϕ1eH1Q−1(θ,ϕ)e1=argmaxθ,ϕeH1Q−1(θ,ϕ)e1 | (42) |
Given azimuth-elevation estimations obtained by mapping the
P(ˆθi,ˆϕi,r) =1aHt,r(ˆθi,ˆϕi,r)UnUHnat,r(ˆθi,ˆϕi,r) | (43) |
For conformal array, different array layouts produce different element patterns. We select the semi conical conformal array which is shown in Fig. 2 as the receiving array for the following simulation.
The simulation parameters are provided as follows:
We first analyze the computational complexity of the algorithms in respect of the calculation of covariance matrix, the eigenvalue decomposition of the matrix and the spectral search. The main complexity of the MUISC algorithm and our proposed RD-MUISC algorithm are respectively as
O(KL(MN)2+4/3(MN)3+Lη1η2η3(MN)2) | (44) |
O(KL(MN)2+4/3(MN)3+Lη1η2(MN)2+Lη3(MN)2) | (45) |
Where
From Eq. (44) and Eq. (45), we can see that the main complexity reduction of the RD-MUSIC algorithm lies in the calculation of the spectral search function. With the increase of the search accuracy, the complexity reduction is more significant.
The computational complexity of algorithms is compared in Fig. 3. It can be seen from Fig. 3 that the difference of computational complexity between the two algorithms gradually increases with the increase of search accuracy. In the case of high accuracy, the computational efficiency of RD-MUSIC algorithm can reach more than
In order to illustrate the effectiveness of the RD-MUSIC algorithm for a single target which is located at
Then, we consider the single target parameter estimation performance, Fig. 5 shows the RMSE of different algorithms with the increase of SNR under 200 snapshots condition, and Fig. 6 demonstrates the RMSE of different algorithms with the increase of snapshot number when SNR=0 dB. As shown in Fig. 5 and Fig. 6, the RMSEs of conformal FDA-MIMO gradually descend with the increasing of SNRs and snapshots, respectively. At the same time, the performance of traditional algorithm is slightly higher than RD-MUSIC algorithm. When the number of snapshots is more than 200, the difference of RMSEs is less than
Without loss of generality, we finally consider two targets which are located at
It can be seen from Fig. 7 that the RMSE curve trend of angle estimation is consistent with that of single target case. The performance of traditional MUSIC algorithm is slightly better than that of RD-MUSIC algorithm. In the range dimension, the performance of traditional algorithm hardly changes with SNR, and RD-MUSIC algorithm is obviously better than traditional MUSIC algorithm. The proposed RD-MUSIC algorithm first estimates the angles, and then estimates the multiple peaks from range-dimensional spectrum, which avoids the ambiguity in the three-dimensional spectral search. Therefore, the RD-MUSIC algorithm has better range resolution for multiple targets estimation.
In this paper, a conformal FDA-MIMO radar is first established, and the corresponding signal receiving mathematical model is formulated. In order to avoid the computational complexity caused by three-dimensional parameter search of MUSIC algorithm, we propose a RD-MUSIC algorithm by solving a quadratic programming problem. Simulation results show that the RD-MUSIC algorithm has comparative angle estimation performance with that of traditional MUSIC algorithm while greatly reducing the computation time. And the RD-MUSIC algorithm has better range estimation performance for multiple targets.
[1] |
何友, 关键, 孟祥伟. 雷达目标检测与恒虚警处理[M]. 2版. 北京: 清华大学出版社, 2011: 1–15.
HE You, GUAN Jian, and MENG Xiangwei. Radar Target Detection and CFAR Processing[M]. 2nd ed. Beijing: Tsinghua University Press, 2011: 1–15.
|
[2] |
黄勇, 陈小龙, 关键. 实测海尖峰特性分析及抑制方法[J]. 雷达学报, 2015, 4(3): 334–342. doi: 10.12000/JR14108
HUANG Yong, CHEN Xiaolong, and GUAN Jian. Property analysis and suppression method of real measured sea spikes[J]. Journal of Radars, 2015, 4(3): 334–342. doi: 10.12000/JR14108
|
[3] |
TRUNK G V and GEORGE S F. Detection of targets in non-Gaussian sea clutter[J]. IEEE Transactions on Aerospace and Electronic Systems, 1970, AES-6(5): 620–628. doi: 10.1109/TAES.1970.310062
|
[4] |
刘宁波, 董云龙, 王国庆, 等. X波段雷达对海探测试验与数据获取[J]. 雷达学报, 2019, 8(5): 656–667. doi: 10.12000/JR19089
LIU Ningbo, DONG Yunlong, WANG Guoqing, et al. Sea-detecting X-band radar and data acquisition program[J]. Journal of Radars, 2019, 8(5): 656–667. doi: 10.12000/JR19089
|
[5] |
YU Xiaohan, CHEN Xiaolong, HUANG Yong, et al. Fast detection method for low-observable maneuvering target via robust sparse fractional Fourier transform[J]. IEEE Geoscience and Remote Sensing Letters, 2020, 17(6): 978–982. doi: 10.1109/LGRS.2019.2939264
|
[6] |
许述文, 石星宇, 水鹏朗. 复合高斯杂波下抑制失配信号的自适应检测器[J]. 雷达学报, 2019, 8(3): 326–334. doi: 10.12000/JR19030
XU Shuwen, SHI Xingyu, and SHUI Penglang. An adaptive detector with mismatched signals rejection in compound Gaussian clutter[J]. Journal of Radars, 2019, 8(3): 326–334. doi: 10.12000/JR19030
|
[7] |
LIU Yi, ZHANG Shufang, SUO Jidong, et al. Research on a new Comprehensive CFAR (Comp-CFAR) processing method[J]. IEEE Access, 2019, 7: 19401–19413. doi: 10.1109/ACCESS.2019.2897358
|
[8] |
WANG H and CAI L. A localized adaptive MTD processor[J]. IEEE Transactions on Aerospace and Electronic Systems, 1991, 27(3): 532–539. doi: 10.1109/7.81435
|
[9] |
CHEN Xiaolong, GUAN Jian, WANG Guoqing, et al. Fast and refined processing of radar maneuvering target based on hierarchical detection via sparse fractional representation[J]. IEEE Access, 2019, 7: 149878–149889. doi: 10.1109/ACCESS.2019.2947169
|
[10] |
CHEN Xiaolong, YU Xiaohan, HUANG Yong, et al. Adaptive clutter suppression and detection algorithm for radar maneuvering target with high-order motions via sparse fractional ambiguity function[J]. IEEE Journal of Selected Topics in Applied Earth Observations and Remote Sensing, 2020, 13: 1515–1526. doi: 10.1109/JSTARS.2020.2981046
|
[11] |
王俊, 郑彤, 雷鹏, 等. 深度学习在雷达中的研究综述[J]. 雷达学报, 2018, 7(4): 395–411. doi: 10.12000/JR18040
WANG Jun, ZHENG Tong, LEI Peng, et al. Study on deep learning in radar[J]. Journal of Radars, 2018, 7(4): 395–411. doi: 10.12000/JR18040
|
[12] |
牟效乾, 陈小龙, 苏宁远, 等. 基于时频图深度学习的雷达动目标检测与分类[J]. 太赫兹科学与电子信息学报, 2019, 17(1): 105–111. doi: 10.11805/TKYDA201901.0105
MOU Xiaoqian, CHEN Xiaolong, SU Ningyuan, et al. Radar detection and classification of moving target using deep convolutional neural networks on time-frequency graphs[J]. Journal of Terahertz Science and Electronic Information Technology, 2019, 17(1): 105–111. doi: 10.11805/TKYDA201901.0105
|
[13] |
REN Shaoqing, HE Kaiming, GIRSHICK R, et al. Faster R-CNN: Towards real-time object detection with region proposal networks[J]. IEEE Transactions on Pattern Analysis and Machine Intelligence, 2015, 39(6): 1137–1149. doi: 10.1109/TPAMI.2016.2577031
|
[14] |
REDMON J and FARHADI A. YOLOv3: An incremental improvement[EB/OL]. https://arxiv.org/abs/1804.02767, 2018.
|
[15] |
BOCHKOVSKIY A, WANG C Y, and LIAO H Y M. YOLOv4: Optimal speed and accuracy of object detection[EB/OL]. https://arxiv.org/abs/2004.10934,2020.
|
[16] |
杜兰, 王兆成, 王燕, 等. 复杂场景下单通道SAR目标检测及鉴别研究进展综述[J]. 雷达学报, 2020, 9(1): 34–54. doi: 10.12000/JR19104
DU Lan, WANG Zhaocheng, WANG Yan, et al. Survey of research progress on target detection and discrimination of single-channel SAR images for complex scenes[J]. Journal of Radars, 2020, 9(1): 34–54. doi: 10.12000/JR19104
|
[17] |
苏宁远, 陈小龙, 陈宝欣, 等. 雷达海上目标双通道卷积神经网络特征融合智能检测方法[J]. 现代雷达, 2019, 41(10): 47–52, 57. doi: 10.16592/j.cnki.1004-7859.2019.10.009
SU Ningyuan, CHEN Xiaolong, CHEN Baoxin, et al. Dual-channel convolutional neural networks feature fusion method for radar maritime target intelligent detection[J]. Modern Radar, 2019, 41(10): 47–52, 57. doi: 10.16592/j.cnki.1004-7859.2019.10.009
|
[18] |
苏宁远, 陈小龙, 关键, 等. 基于卷积神经网络的海上微动目标检测与分类方法[J]. 雷达学报, 2018, 7(5): 565–574. doi: 10.12000/JR18077
SU Ningyuan, CHEN Xiaolong, GUAN Jian, et al. Detection and classification of maritime target with micro-motion based on CNNs[J]. Journal of Radars, 2018, 7(5): 565–574. doi: 10.12000/JR18077
|
[19] |
CHEN Chen, HE Chuan, HU Changhua, et al. MSARN: A deep neural network based on an adaptive recalibration mechanism for multiscale and arbitrary-oriented SAR ship detection[J]. IEEE Access, 2019, 7: 159262–159283. doi: 10.1109/ACCESS.2019.2951030
|
[20] |
黄洁, 姜志国, 张浩鹏, 等. 基于卷积神经网络的遥感图像舰船目标检测[J]. 北京航空航天大学学报, 2017, 43(9): 1841–1848. doi: 10.13700/j.bh.1001-5965.2016.0755
HUANG Jie, JIANG Zhiguo, ZHANG Haopeng, et al. Ship object detection in remote sensing images using convolutional neural networks[J]. Journal of Beijing University of Aeronautics and Astronautics, 2017, 43(9): 1841–1848. doi: 10.13700/j.bh.1001-5965.2016.0755
|
[21] |
WEI Xiukun, WEI Dehua, SUO Da, et al. Multi-target defect identification for railway track line based on image processing and improved YOLOv3 model[J]. IEEE Access, 2020, 8: 61973–61988. doi: 10.1109/ACCESS.2020.2984264
|
[22] |
XIAO Dong, SHAN Feng, LI Ze, et al. A target detection model based on improved Tiny-Yolov3 under the environment of mining truck[J]. IEEE Access, 2019, 7: 123757–123764. doi: 10.1109/ACCESS.2019.2928603
|
[23] |
ZHANG Huibing, QIN Longfei, LI Jun, et al. Real-time detection method for small traffic signs based on Yolov3[J]. IEEE Access, 2020, 8: 64145–64156. doi: 10.1109/ACCESS.2020.2984554
|
[24] |
BA J L, KIROS J R, and HINTON G E. Layer normalization[EB/OL]. https://arxiv.org/abs/1607.06450, 2016.
|
[25] |
WANG C Y, LIAO H Y M, YEH I H, et al. CSPNet: A new backbone that can enhance learning capability of CNN[EB/OL]. https://arxiv.org/abs/1911.11929,2019.
|
[26] |
HE Kaiming, ZHANG Xiangyu, REN Shaoqing, et al. Deep residual learning for image recognition[C]. 2016 IEEE Conference on Computer Vision and Pattern Recognition (CVPR), Las Vegas, USA, 2016: 770–778. doi: 10.1109/CVPR.2016.90.
|
[27] |
AI Jiaqiu, YANG Xuezhi, DONG Zhangyu, et al. A new two parameter CFAR ship detector in Log-Normal clutter[C]. 2017 IEEE Radar Conference (RadarConf), Seattle, USA, 2017: 195–199. doi: 10.1109/RADAR.2017.7944196.
|
[28] |
YANG Jianyu, LU Chao, and LI Liangchao. Target detection in passive millimeter wave image based on two-dimensional cell-weighted average CFAR[C]. The IEEE 11th International Conference on Signal Processing, Beijing, China, 2012: 917–921. doi: 10.1109/ICoSP.2012.6491729.
|
1. | 葛津津,周浩,凌天庆. 一种应用于脉冲探地雷达前端的探测子系统. 电子测量技术. 2022(04): 27-32 . ![]() | |
2. | 尹诗,郭伟. 用于探地雷达的超宽带天线设计与仿真. 电子设计工程. 2018(03): 98-102 . ![]() | |
3. | 宋立伟,张超,洪涛. 冲击波载荷对平面阵列天线电性能的影响. 电子机械工程. 2017(04): 1-5+58 . ![]() | |
4. | 尹德,叶盛波,刘晋伟,纪奕才,刘小军,方广有. 一种用于高速公路探地雷达的新型时域超宽带TEM喇叭天线. 雷达学报. 2017(06): 611-618 . ![]() |