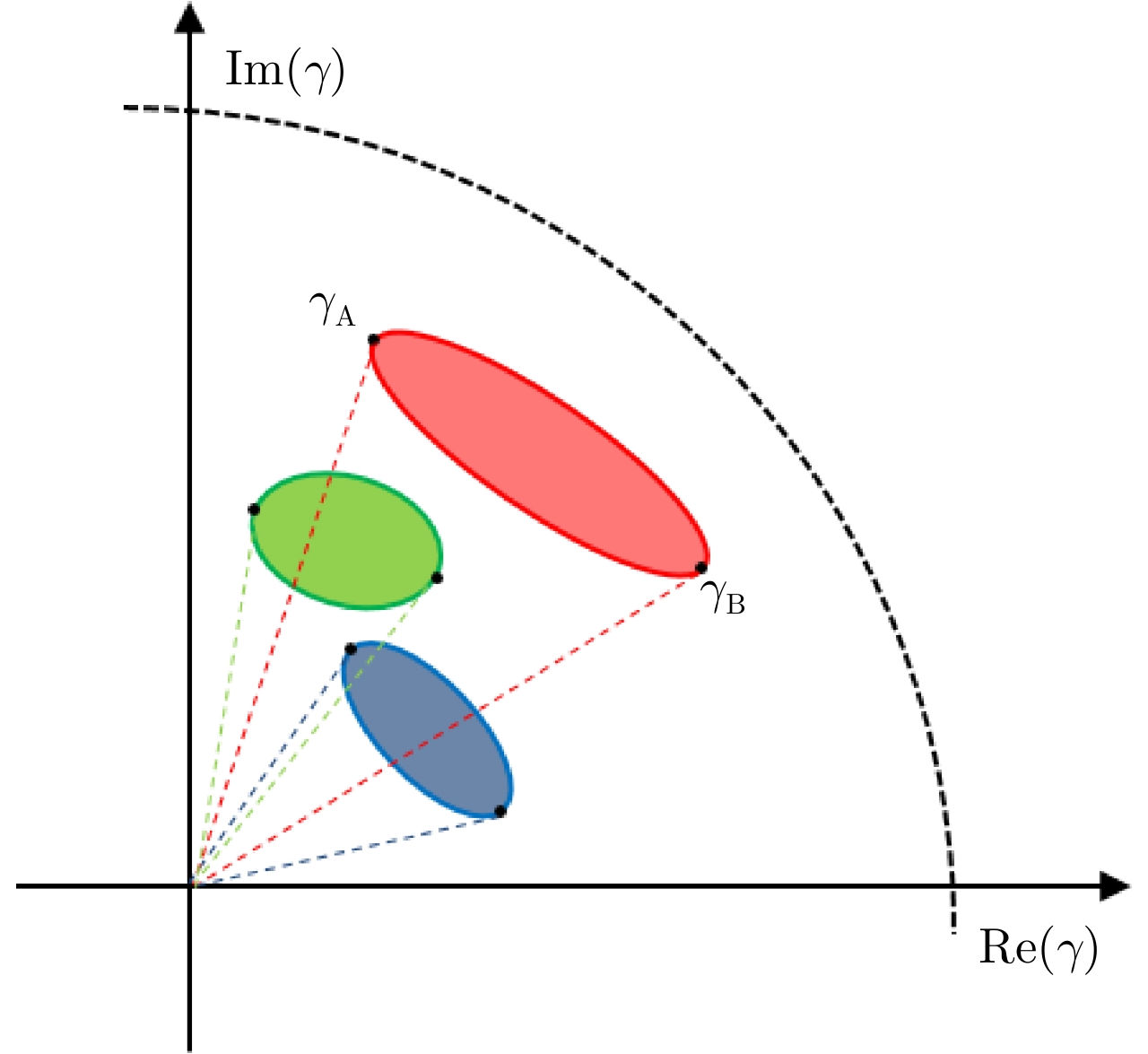
Citation: | GUO Wenjie, WU Zhenhua, CAO Yice, et al. Multidomain characteristic-guided multimodal contrastive recognition method for active radar jamming[J]. Journal of Radars, 2024, 13(5): 1004–1018. doi: 10.12000/JR24129 |
森林高度是估算森林蓄积量及生物量的重要基础数据,对于研究森林资源状况以及分析全球生态环境、气候变化具有重要意义。极化合成孔径雷达干涉测量技术(Polarimetric SAR Interferometry, PolInSAR)采用微波监测模式,其回波信号不仅记录垂直结构及其属性信息,且可以区分同一分辨单元内不同散射体高度的能力,已被视为大范围、高分辨率、高精度反演森林高度的有效手段之一[1]。
为了实现利用PolInSAR观测量准确地提取森林高度,Thrauhft等人[2]建立了随机地体二层散射模型(Random Volume over Ground, RVoG),该模型将森林散射场景抽象为两层,即由随机均匀分布的散射体组成的植被层,以及微波信号不可穿透的地表层。随后,Papathanassiou等人[3,4]进一步分析PolInSAR复相干性与RVoG模型的关联,建立了利用PolInSAR反演森林高度的框架。实质上,该框架是基于体散射去相干的模型表达来反演森林高度等参数的,并且利用不同PolInSAR数据都获得了较高的反演精度[5-7]。
由于森林场景具有显著的时变性,具有长时间间隔的星载重轨干涉SAR(如ALOS-1至少为46天,ALOS-2为14天)散射场景内介电常数变化(如降雨)和风动都会产生严重的时间去相干。因此,除了体去相干的影响,时间去相干也是星载重轨极化干涉SAR数据中不可忽略的去相干因素,决定了森林参数反演的精度,甚至是反演成败的关键。为此,Yang等人[8]在随机移动散射模型(Random Motion over Ground, RMoG)[9]和体时去相干散射模型 (Volume Temporal Decorrelation, VTD)模型[10]基础上,提出一种时间去相干半经验森林高度反演方法。该方法结合少量机载LiDAR森林高度数据辅助时间去相干半经验模型解算,利用ALOS-1 PARSAR-1 HV极化相干幅度成功实现了大尺度森林高度反演。
然而,该方法需假设HV极化不包含地表散射回波能量贡献,事实上,L波段SAR信号具有较强的穿透性,尤其当森林高度较低或密度较小时,HV极化方式会记录显著地表回波信号。此外,该方法只适用于单基线干涉数据,尚未考虑多基线条件下,如何充分利用观测几何的多样性提升反演结果的可靠性。因此本文的目的是针对上述反演方法的限制,利用ALOS-2 PARSAR-2多基线PolInSAR数据更为准确地提取森林高度。主要思路如下:首先利用相干最大分离算法(Maximum Coherence Difference, MCD)在极化空间内寻求具有最少地面散射能量贡献的极化方式,以获得更为纯净的森林冠层散射贡献。然后利用该极化方式的相干幅度,在少量森林高度地面调查数据辅助下基于时间去相干半经验模型进行森林高度反演。在此基础之上,结合多基线数据根据PolInSAR相干集在复数平面内的几何表达,甄选最优观测干涉数据的反演结果作为森林高度反演最终结果。
综合顾及垂直方向上散射体分布产生的体去相干、散射场景内介电特性改变和植被风动引起的时间去相干同时占主导地位,星载重轨PolInSAR复相干系数一般形式表示为[8]
γ(ω)=eiφ0⋅γvd⋅γv/m+γgd⋅μ(ω)1+μ(ω) | (1) |
式中,
γv/m=∫h0exp[−12(4πλ)2σ2r(z)]⋅f(z)⋅exp(ikzz)dz∫h0f(z)dz | (2) |
其中,
σr(z)=σrhrz | (3) |
式中,
为了解决上述模型过参数化问题,Yang等人[8]对散射场景做如下假设:(1) 散射场景内时间去相干与消光系数在空间上具有一致性;(2) HV极化方式具有较小地面散射能量贡献,可假定其地体幅度比
γ=Sscene⋅exp[−12(4πσrαλhr)2h2]≈Sscene⋅sinc(hCscene) | (4) |
其中,
Sscene=|γvd|; Cscene=λhr2π2σrα | (5) |
已有方法主要选用对森林冠层较为敏感的HV极化方式进行模型求解,但是ALOS-2 PALSAR-2发射具有较强穿透能力的L波段电磁波,HV极化方式回波信号中同样会记录显著地表回波信号。鉴于此,本文利用ALOS-2 PALSAR-2全极化数据结合极化相干最优理论,尽可能抑制地表回波信号的干扰。具体方法如下:
在主辅极化SAR影像散射机制相同的情况下,极化干涉SAR的复相干系数表示为[12]
γ=⟨ωHΩ12ω⟩√⟨ωHT11ω⟩⟨ωHT22ω⟩ | (6) |
式中,自相关矩阵
Re(γeiϕ)=ωHAωωHTωA=eiϕΩ12+e−iϕΩH122,T=T11+T222} | (7) |
式中,
γ1=ωH1Ω12ω1ωH1Tω1, γ2=ωH2Ω12ω2ωH2Tω2 | (8) |
相比传统InSAR技术只能获取HH, HV或VV极化方式对应的复相干系数,相干集中包含了特定极化散射机理对应的复相干系数,为寻求极化空间内具有更为纯净森林冠层散射贡献的极化方式提供了可能。相干区域范围示意如图1所示,其中在相干区域成对边界点中距离最远的一对相干系数点
kz>0:ifarg(γAγ∗B)>0thenγ(μmin)=γA,γ(μmax)=γBifarg(γAγ∗B)<0thenγ(μmin)=γB,γ(μmax)=γAkz<0:ifarg(γAγ∗B)<0thenγ(μmin)=γA,γ(μmax)=γBifarg(γAγ∗B)>0thenγ(μmin)=γB,γ(μmax)=γA} | (9) |
其中,
kz=4πB⊥λRsinθ | (10) |
即便简化了模型参数和采用多基线PolInSAR数据增加了观测量,利用传统多维非线性迭代求解时间去相干半经验模型仍存在秩亏问题。因此本文采用一种外部数据辅助反演法[8],即先利用小范围真实森林高度数据辅助解算出模型参数
主成分分析思想[17]为实现上述思路提供了契机,即通过对训练数据中
X=[Var(hreal)Cov(hreal,hinvert)Cov(hinvert,hreal)Var(hinvert)]=[P11P12P21P22][λ100λ2][P11P12P21P22]−1k=P21P11} | (11) |
其中
b=M(hreal)−M(hinvert)[M(hreal)+M(hinvert)]/2 | (12) |
式中,M 表示取平均运算。
散点点阵椭圆主轴确定后,显然可以通过建立使逼近参数
(k−1)2+(b−0)2=min | (13) |
该目标函数可以利用高斯-牛顿迭代算法进行非线性最小二乘求解,如式(14)所示
[S∗sceneC∗scene]=(JT0J0)−1JT0[1−k00−b0]+[Sscene0Cscene0] | (14) |
式中,
J0=[∂k∂Sscene∂k∂Cscene∂b∂Sscene∂b∂Cscene]|Sscene0Cscene0 | (15) |
然后将得到修正后的模型参数作为新的初始点进行下一次迭代,经过多次迭代后即可获得最佳模型参数
|[S∗sceneC∗scene]−[S(∗−1)sceneC(∗−1)scene]|<ε | (16) |
在利用训练数据求得时间去相干半经验模型参数后,对每个像元求解一元非线性方程得到整个散射场景内的森林高度结果。
时间去相干、体去相干以及其他噪声等因素会共同影响PolInSAR复相干性在复平面单位圆上的几何表达[18]。在多基线配置下,不同干涉对在同一分辨单元内往往呈现出不同的相干区域结构(如图1所示)。而相干特性
P=|γ(μmin)−γ(μmax)||γ(μmin)+γ(μmax)| | (17) |
式中,
通过相干特性指标P 甄选出不同干涉对在同一分辨单元内反演出的最优森林高度值作为多基线PolInSAR森林高度融合结果,多基线PolInSAR融合反演框架可表示为
max‖P1(γ1(μmin),γ1(μmax))P2(γ2(μmin),γ2(μmax))⋮PN(γN(μmin),γN(μmax))‖ | (18) |
式中,N 为极化干涉SAR观测基线数。
研究区域黄丰桥国有林场(27°
地面实测数据由中南林业科技大学于2016年6~7月采集得到,通过在林区范围内选取60个相互独立的林分样地以确保避免空间自相关,每个林分样地规格为30×30 m。树高则基于单木测高原理利用激光测高仪测得,林分高度范围为4.60~20.20 m,平均高度为13.24 m。本文通过随机采样,将60个林分样地数据随机分为45个训练数据(图3黄点所示)和15个验证数据(图3红点所示)两组。
多基线星载重轨PolInSAR数据是利用日本宇航局(JAXA)提供的5景覆盖研究区域的ALOS-2 PALSAR-2 L波段全极化数据。该SAR影像范围如图3蓝色虚线所示,获取时间为2016年6月至8月,获取模式为StripMap2(SM2),影像主要参数信息如表1所示。将5景SAR影像组成3个时间基线为14天的干涉影像对(BL1, BL2, BL3),然后各自进行配准处理,并进行公共带通滤波以确保去除几何去相干。相干性以11×11窗口进行估计,并应用Boxcar滤波进行平滑处理以消除斑点效应。最后利用SRTM DEM对SAR影像进行地理编码,并将其重采样至与DEM空间分辨率一致(30×30 m)。图4为3个干涉对的HV极化和
日期(2016年) | 垂直有效波数(rad/m) | 时间基线(天) | 距离向/方位向分辨率(m) | 中心入射角 (°) | 极化方式 |
0616—0630 (BL1) | 0.013~0.015 | ||||
0630—0714 (BL2) | 0.010~0.011 | 14 | 2.86/2.97 | 38.99 | Full |
0811—0825 (BL3) | 0.009~0.010 |
以选取的15个验证林分的实测森林高度(H-field)对反演结果(H-invert)进行分析评价,图5为3个干涉对利用HV极化反演得到的散点图结果,均方根误差RMSE分别为:4.20 m, 4.03 m和3.42 m。利用
从上述单基线森林高度反演结果看,不同干涉对反演整体精度较为接近,但是对于同一林分在利用不同干涉对反演的结果却存在明显差异。因此,当多基线数据可用时,我们进一步在单基线PolInSAR森林高度反演结果的基础上挖掘PolInSAR数据本身特性并对其森林高度反演能力进行评判。与时间去相干相关的参数
模型参数 | BL1 | BL2 | BL3 |
Sscene | 0.69 | 0.78 | 0.78 |
Cscene | 9.88 | 10.08 | 11.14 |
3个干涉对在验证林分的相干特性P值、反演森林高度值以及多基线融合森林高度值如表3所示,从整体看,根据相干特性P值大小从3个单基线PolInSAR反演结果中甄选出的森林高度结果更接近于实测真实森林高度。整个实验区的多基线PolInSAR融合反演结果以及精度评定如图7所示,均方根误差RMSE为2.05 m,相比于已有的方法,本文提出的多基线PolInSAR融合反演策略精度至少提高了40%(与图5中BL3基线结果对比),同时,相关系数也提升至0.81。
林分样地编号 | BL1 P值 / 森林高度(m) | BL2 P值 / 森林高度(m) | BL3 P值 / 森林高度(m) | 多基线融合结果(m) | 实测森林高度(m) |
1 | 0.130 / 17.82 | 0.113 / 17.02 | 0.081 / 16.89 | 17.82 | 14.43 |
2 | 0.116 / 14.38 | 0.104 / 15.30 | 0.091 / 16.52 | 14.38 | 14.20 |
3 | 0.092 / 12.46 | 0.075 / 15.83 | 0.135 / 11.34 | 11.34 | 9.80 |
4 | 0.103 / 15.21 | 0.111 / 15.34 | 0.119 / 14.19 | 14.19 | 16.00 |
5 | 0.106 / 6.86 | 0.106 / 7.24 | 0.131 / 8.31 | 8.31 | 10.70 |
6 | 0.110 / 12.98 | 0.083 / 14.67 | 0.118 / 11.89 | 11.89 | 13.50 |
7 | 0.114 / 13.35 | 0.096 / 15.30 | 0.101 / 16.10 | 13.35 | 13.43 |
8 | 0.079 / 14.29 | 0.106 / 16.15 | 0.117 / 16.22 | 16.22 | 16.95 |
9 | 0.069 / 12.12 | 0.090 / 17.63 | 0.060 / 12.30 | 17.63 | 20.10 |
10 | 0.104 / 12.33 | 0.089 / 13.67 | 0.102 / 11.72 | 12.33 | 15.60 |
11 | 0.075 / 18.34 | 0.103 / 16.75 | 0.154 / 10.16 | 10.16 | 13.30 |
12 | 0.113 / 9.08 | 0.134 / 9.46 | 0.106 / 12.69 | 9.46 | 11.00 |
13 | 0.086 / 13.76 | 0.096 / 9.07 | 0.109 / 16.00 | 16.00 | 16.40 |
14 | 0.197 / 10.17 | 0.230 / 8.71 | 0.186 / 9.51 | 8.71 | 6.00 |
15 | 0.103 / 14.59 | 0.064 / 19.17 | 0.128 / 15.40 | 15.40 | 14.70 |
在多基线全极化数据可用条件下,弥补单基线InSAR观测信息不足以及几何结构单一的问题,对于反演结果整体精度提升具有重要作用。本文提出了一种星载重轨多基线PolInSAR反演森林高度的策略,对InSAR极化空间和观测几何空间进行扩展,主要结论如下:
(1) 该方法利用MCD相干优化算法获得对体散射最为敏感的极化方式,并基于时间去相干半经验模型进行森林高度反演,使每条单基线反演精度在一定程度上都有所提高。
(2) 利用由相干特性指标P确定的相干区域最优准则可以优选出同一分辨单元内最优的单基线森林高度反演结果。因此,相比仅利用单基线单一极化反演方法,多基线PolInSAR融合策略具有更好的稳定性,精度也更高。
[1] |
崔国龙, 余显祥, 魏文强, 等. 认知智能雷达抗干扰技术综述与展望[J]. 雷达学报, 2022, 11(6): 974–1002. doi: 10.12000/JR22191.
CUI Guolong, YU Xianxiang, WEI Wenqiang, et al. An overview of antijamming methods and future works on cognitive intelligent radar[J]. Journal of Radars, 2022, 11(6): 974–1002. doi: 10.12000/JR22191.
|
[2] |
解烽, 刘环宇, 胡锡坤, 等. 基于复数域深度强化学习的多干扰场景雷达抗干扰方法[J]. 雷达学报, 2023, 12(6): 1290–1304. doi: 10.12000/JR23139.
XIE Feng, LIU Huanyu, HU Xikun, et al. A radar anti-jamming method under multi-jamming scenarios based on deep reinforcement learning in complex domains[J]. Journal of Radars, 2023, 12(6): 1290–1304. doi: 10.12000/JR23139.
|
[3] |
周红平, 王子伟, 郭忠义. 雷达有源干扰识别算法综述[J]. 数据采集与处理, 2022, 37(1): 1–20. doi: 10.16337/j.1004-9037.2022.01.001.
ZHOU Hongping, WANG Ziwei, and GUO Zhongyi. Overview on recognition algorithms of radar active jamming[J]. Journal of Data Acquisition and Processing, 2022, 37(1): 1–20. doi: 10.16337/j.1004-9037.2022.01.001.
|
[4] |
LV Qinzhe, FAN Hanxin, LIU Junliang, et al. Multilabel deep learning-based lightweight radar compound jamming recognition method[J]. IEEE Transactions on Instrumentation and Measurement, 2024, 73: 2521115. doi: 10.1109/TIM.2024.3400337.
|
[5] |
CAO Ru, CAO Jiuwen, MEI Jianping, et al. Radar emitter identification with bispectrum and hierarchical extreme learning machine[J]. Multimedia Tools and Applications, 2019, 78(20): 28953–28970. doi: 10.1007/s11042-018-6134-y.
|
[6] |
QU Qizhe, WANG Yongliang, LIU Weijian, et al. IRNet: Interference recognition networks for automotive radars via autocorrelation features[J]. IEEE Transactions on Microwave Theory and Techniques, 2022, 70(5): 2762–2774. doi: 10.1109/TMTT.2022.3151635.
|
[7] |
WU Zhenhua, QIAN Jun, ZHANG Man, et al. High-confidence sample augmentation based on label-guided denoising diffusion probabilistic model for active deception jamming recognition[J]. IEEE Geoscience and Remote Sensing Letters, 2023, 20: 3508305. doi: 10.1109/LGRS.2023.3316282.
|
[8] |
ZOU Wenxu, XIE Kai, and LIN Jinjian. Light-weight deep learning method for active jamming recognition based on improved MobileViT[J]. IET Radar, Sonar & Navigation, 2023, 17(8): 1299–1311. doi: 10.1049/rsn2.12420.
|
[9] |
LV Qinzhe, QUAN Yinghui, FENG Wei, et al. Radar deception jamming recognition based on weighted ensemble CNN with transfer learning[J]. IEEE Transactions on Geoscience and Remote Sensing, 2022, 60: 5107511. doi: 10.1109/TGRS.2021.3129645.
|
[10] |
LUO Zhenyu, CAO Yunhe, YEO T S, et al. Few-shot radar jamming recognition network via time-frequency self-attention and global knowledge distillation[J]. IEEE Transactions on Geoscience and Remote Sensing, 2023, 61: 5105612. doi: 10.1109/TGRS.2023.3280322.
|
[11] |
KONG Yukai, XIA Senlin, DONG Luxin, et al. Compound jamming recognition via contrastive learning for distributed MIMO radars[J]. IEEE Transactions on Vehicular Technology, 2024, 73(6): 7892–7907. doi: 10.1109/TVT.2024.3358996.
|
[12] |
KONG Yukai, WANG Xiang, WU Changxin, et al. Active deception jamming recognition in the presence of extended target[J]. IEEE Geoscience and Remote Sensing Letters, 2022, 19: 4024905. doi: 10.1109/LGRS.2022.3184997.
|
[13] |
ZHOU Hongping, WANG Lei, and GUO Zhongyi. Recognition of radar compound jamming based on convolutional neural network[J]. IEEE Transactions on Aerospace and Electronic Systems, 2023, 59(6): 7380–7394. doi: 10.1109/TAES.2023.3288080.
|
[14] |
PENG Ruihui, WEI Wenbin, SUN Dianxing, et al. Dense false target jamming recognition based on fast-slow time domain joint frequency response features[J]. IEEE Transactions on Aerospace and Electronic Systems, 2023, 59(6): 9142–9159. doi: 10.1109/TAES.2023.3316125.
|
[15] |
魏赓力, 李凉海, 闫海鹏, 等. 基于多模特征融合的雷达干扰信号识别[J]. 遥测遥控, 2023, 44(4): 80–87. doi: 10.12347/j.ycyk.20230328001.
WEI Gengli, LI Lianghai, YAN Haipeng, et al. Radar jamming signal identification based on multimode feature fusion[J]. Journal of Telemetry, Tracking and Command, 2023, 44(4): 80–87. doi: 10.12347/j.ycyk.20230328001.
|
[16] |
张顺生, 陈爽, 陈晓莹, 等. 面向小样本的多模态雷达有源欺骗干扰识别方法[J]. 雷达学报, 2023, 12(4): 882–891. doi: 10.12000/JR23104.
ZHANG Shunsheng, CHEN Shuang, CHEN Xiaoying, et al. Active deception jamming recognition method in multimodal radar based on small samples[J]. Journal of Radars, 2023, 12(4): 882–891. doi: 10.12000/JR23104.
|
[17] |
SHAO Guangqing, CHEN Yushi, and WEI Yinsheng. Deep fusion for radar jamming signal classification based on CNN[J]. IEEE Access, 2020, 8: 117236–117244. doi: 10.1109/ACCESS.2020.3004188.
|
[18] |
ZHANG Yipeng, LU Dongdong, QIU Xiaolan, et al. Scattering-point topology for few-shot ship classification in SAR images[J]. IEEE Journal of Selected Topics in Applied Earth Observations and Remote Sensing, 2023, 16: 10326–10343. doi: 10.1109/JSTARS.2023.3328066.
|
[19] |
GENG Jie, ZHANG Yuhang, and JIANG Wen. Polarimetric SAR image classification based on hierarchical scattering-spatial interaction transformer[J]. IEEE Transactions on Geoscience and Remote Sensing, 2024, 62: 5205014. doi: 10.1109/TGRS.2024.3362360.
|
[20] |
郭帅, 陈婷, 王鹏辉, 等. 基于角度引导Transformer融合网络的多站协同目标识别方法[J]. 雷达学报, 2023, 12(3): 516–528. doi: 10.12000/JR23014.
GUO Shuai, CHEN Ting, WANG Penghui, et al. Multistation cooperative radar target recognition based on an angle-guided Transformer fusion network[J]. Journal of Radars, 2023, 12(3): 516–528. doi: 10.12000/JR23014.
|
[21] |
CHEN Xiaolong, SU Ningyuan, HUANG Yong, et al. False-alarm-controllable radar detection for marine target based on multi features fusion via CNNs[J]. IEEE Sensors Journal, 2021, 21(7): 9099–9111. doi: 10.1109/JSEN.2021.3054744.
|
[22] |
RADFORD A, KIM J W, HALLACY C, et al. Learning transferable visual models from natural language supervision[C]. The 38th International Conference on Machine Learning (ICML), 2021: 8748–8763.
|
[23] |
李宏, 郑光勇, 郭雷, 等. 雷达相参压制干扰信号分析[J]. 电子信息对抗技术, 2013, 28(3): 36–40. doi: 10.3969/j.issn.1674-2230.2013.03.009.
LI Hong, ZHENG Guangyong, GUO Lei, et al. Jamming analysis of radar coherent suppressing[J]. Electronic Information Warfare Technology, 2013, 28(3): 36–40. doi: 10.3969/j.issn.1674-2230.2013.03.009.
|
[24] |
WEI Shaopeng, ZHANG Lei, LU Jingyue, et al. Modulated-ISRJ rejection using online dictionary learning for synthetic aperture radar imagery[J]. Journal of Systems Engineering and Electronics, 2024, 35(2): 316–329. doi: 10.23919/JSEE.2023.000076.
|
[25] |
TAN Mingxing and LE Q V. EfficientNetV2: Smaller models and faster training[C]. The 38th International Conference on Machine Learning (ICML), 2021: 10096–10106.
|
[26] |
GONG Heng, FENG Xiaocheng, QIN Bing, et al. Table-to-text generation with effective hierarchical encoder on three dimensions (row, column and time)[C]. The 2019 Conference on Empirical Methods in Natural Language Processing and the 9th International Joint Conference on Natural Language Processing, Hong Kong, China, 2019: 3143–3152. doi: 10.18653/v1/D19-1310.
|
[27] |
LI Liang, MA Can, YUE Yinliang, et al. Improving encoder by auxiliary supervision tasks for table-to-text generation[C]. The 59th Annual Meeting of the Association for Computational Linguistics and the 11th International Joint Conference on Natural Language Processing (Volume 1: Long Papers), 2021: 5979–5989. doi: 10.18653/v1/2021.acl-long.466.
|
[28] |
RADFORD A, WU J, CHILD R, et al. Language models are unsupervised multitask learners[J]. OpenAI Blog, 2019, 1(8): 9.
|
[29] |
KHOSLA P, TETERWAK P, WANG Chen, et al. Supervised contrastive learning[C]. The 34th International Conference on Neural Information Processing Systems (NIPS’20), Vancouver, Canada, 2020: 1567.
|
[30] |
WANG Feng and LIU Huaping. Understanding the behaviour of contrastive loss[C]. IEEE/CVF Conference on Computer Vision and Pattern Recognition, Nashville, USA, 2021: 2495–2504. doi: 10.1109/CVPR46437.2021.00252.
|
[31] |
LIU Mingqian, LIU Zilong, LU Weidang, et al. Distributed few-shot learning for intelligent recognition of communication jamming[J]. IEEE Journal of Selected Topics in Signal Processing, 2022, 16(3): 395–405. doi: 10.1109/JSTSP.2021.3137028.
|
1. | 汪思源,曲毅,陈怡君. 基于U-Net的涡旋电磁波雷达成像方法. 空军工程大学学报. 2024(03): 77-85 . ![]() | |
2. | 潘浩然,马晖,胡敦法,刘宏伟. 基于涡旋电磁波新体制的雷达前视三维成像. 雷达学报. 2024(05): 1109-1122 . ![]() | |
3. | 毛德庆,杨建宇,杨明杰,张永超,张寅,黄钰林. IAA-Net:一种实孔径扫描雷达迭代自适应角超分辨成像方法. 雷达学报. 2024(05): 1073-1091 . ![]() | |
4. | 马晖,胡敦法,师竹雨,刘宏伟. 基于涡旋电磁波的雷达应用研究进展. 现代雷达. 2023(05): 27-41 . ![]() | |
5. | 袁航,罗迎,陈怡君,苏令华. 基于反正弦圆环天线阵列的二维成像. 北京航空航天大学学报. 2023(06): 1487-1494 . ![]() |
日期(2016年) | 垂直有效波数(rad/m) | 时间基线(天) | 距离向/方位向分辨率(m) | 中心入射角 (°) | 极化方式 |
0616—0630 (BL1) | 0.013~0.015 | ||||
0630—0714 (BL2) | 0.010~0.011 | 14 | 2.86/2.97 | 38.99 | Full |
0811—0825 (BL3) | 0.009~0.010 |
模型参数 | BL1 | BL2 | BL3 |
Sscene | 0.69 | 0.78 | 0.78 |
Cscene | 9.88 | 10.08 | 11.14 |
林分样地编号 | BL1 P值 / 森林高度(m) | BL2 P值 / 森林高度(m) | BL3 P值 / 森林高度(m) | 多基线融合结果(m) | 实测森林高度(m) |
1 | 0.130 / 17.82 | 0.113 / 17.02 | 0.081 / 16.89 | 17.82 | 14.43 |
2 | 0.116 / 14.38 | 0.104 / 15.30 | 0.091 / 16.52 | 14.38 | 14.20 |
3 | 0.092 / 12.46 | 0.075 / 15.83 | 0.135 / 11.34 | 11.34 | 9.80 |
4 | 0.103 / 15.21 | 0.111 / 15.34 | 0.119 / 14.19 | 14.19 | 16.00 |
5 | 0.106 / 6.86 | 0.106 / 7.24 | 0.131 / 8.31 | 8.31 | 10.70 |
6 | 0.110 / 12.98 | 0.083 / 14.67 | 0.118 / 11.89 | 11.89 | 13.50 |
7 | 0.114 / 13.35 | 0.096 / 15.30 | 0.101 / 16.10 | 13.35 | 13.43 |
8 | 0.079 / 14.29 | 0.106 / 16.15 | 0.117 / 16.22 | 16.22 | 16.95 |
9 | 0.069 / 12.12 | 0.090 / 17.63 | 0.060 / 12.30 | 17.63 | 20.10 |
10 | 0.104 / 12.33 | 0.089 / 13.67 | 0.102 / 11.72 | 12.33 | 15.60 |
11 | 0.075 / 18.34 | 0.103 / 16.75 | 0.154 / 10.16 | 10.16 | 13.30 |
12 | 0.113 / 9.08 | 0.134 / 9.46 | 0.106 / 12.69 | 9.46 | 11.00 |
13 | 0.086 / 13.76 | 0.096 / 9.07 | 0.109 / 16.00 | 16.00 | 16.40 |
14 | 0.197 / 10.17 | 0.230 / 8.71 | 0.186 / 9.51 | 8.71 | 6.00 |
15 | 0.103 / 14.59 | 0.064 / 19.17 | 0.128 / 15.40 | 15.40 | 14.70 |
日期(2016年) | 垂直有效波数(rad/m) | 时间基线(天) | 距离向/方位向分辨率(m) | 中心入射角 (°) | 极化方式 |
0616—0630 (BL1) | 0.013~0.015 | ||||
0630—0714 (BL2) | 0.010~0.011 | 14 | 2.86/2.97 | 38.99 | Full |
0811—0825 (BL3) | 0.009~0.010 |
模型参数 | BL1 | BL2 | BL3 |
Sscene | 0.69 | 0.78 | 0.78 |
Cscene | 9.88 | 10.08 | 11.14 |
林分样地编号 | BL1 P值 / 森林高度(m) | BL2 P值 / 森林高度(m) | BL3 P值 / 森林高度(m) | 多基线融合结果(m) | 实测森林高度(m) |
1 | 0.130 / 17.82 | 0.113 / 17.02 | 0.081 / 16.89 | 17.82 | 14.43 |
2 | 0.116 / 14.38 | 0.104 / 15.30 | 0.091 / 16.52 | 14.38 | 14.20 |
3 | 0.092 / 12.46 | 0.075 / 15.83 | 0.135 / 11.34 | 11.34 | 9.80 |
4 | 0.103 / 15.21 | 0.111 / 15.34 | 0.119 / 14.19 | 14.19 | 16.00 |
5 | 0.106 / 6.86 | 0.106 / 7.24 | 0.131 / 8.31 | 8.31 | 10.70 |
6 | 0.110 / 12.98 | 0.083 / 14.67 | 0.118 / 11.89 | 11.89 | 13.50 |
7 | 0.114 / 13.35 | 0.096 / 15.30 | 0.101 / 16.10 | 13.35 | 13.43 |
8 | 0.079 / 14.29 | 0.106 / 16.15 | 0.117 / 16.22 | 16.22 | 16.95 |
9 | 0.069 / 12.12 | 0.090 / 17.63 | 0.060 / 12.30 | 17.63 | 20.10 |
10 | 0.104 / 12.33 | 0.089 / 13.67 | 0.102 / 11.72 | 12.33 | 15.60 |
11 | 0.075 / 18.34 | 0.103 / 16.75 | 0.154 / 10.16 | 10.16 | 13.30 |
12 | 0.113 / 9.08 | 0.134 / 9.46 | 0.106 / 12.69 | 9.46 | 11.00 |
13 | 0.086 / 13.76 | 0.096 / 9.07 | 0.109 / 16.00 | 16.00 | 16.40 |
14 | 0.197 / 10.17 | 0.230 / 8.71 | 0.186 / 9.51 | 8.71 | 6.00 |
15 | 0.103 / 14.59 | 0.064 / 19.17 | 0.128 / 15.40 | 15.40 | 14.70 |